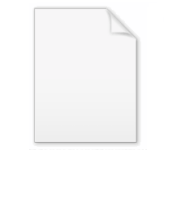
Bilingual evaluation understudy
Encyclopedia
BLEU is an algorithm for evaluating the quality of text which has been machine-translated
from one natural language
to another. Quality is considered to be the correspondence between a machine's output and that of a human: "the closer a machine translation is to a professional human translation, the better it is". BLEU was one of the first metrics
to achieve a high correlation
with human judgements of quality, and remains one of the most popular.
Scores are calculated for individual translated segments—generally sentences—by comparing them with a set of good quality reference translations. Those scores are then averaged over the whole corpus
to reach an estimate of the translation's overall quality. Intelligibility or grammatical correctness are not taken into account.
BLEU is designed to approximate human judgement at a corpus level, and performs badly if used to evaluate the quality of individual sentences.
BLEU’s output is always a number between 0 and 1. This value indicates how similar the candidate and reference texts are, with values closer to 1 representing more similar texts.
Of the seven words in the candidate translation, all of them appear in the reference translations. Thus the candidate text is given a unigram precision of,

where
is number of words from the candidate that are found in the reference, and
is the total number of words in the candidate. This is a perfect score, despite the fact that the candidate translation above retains little of the content of either of the references.
The modification that BLEU makes is fairly straightforward. For each word in the candidate translation, the algorithm takes its maximum total count,
, in any of the reference translations. In the example above, the word "the" appears twice in reference 1, and once in reference 2. Thus
.
For the candidate translation, the count
of each word is clipped to a maximum of
for that word. In this case, "the" has
and
, thus
is clipped to 2.
is then summed over all words in the candidate.
This sum is then divided by the total number of words in the candidate translation. In the above example, the modified unigram precision score would be:

The above method is used to calculate scores for a range of n-gram lengths. The length which has the "highest correlation with monolingual human judgements" was found to be four. The unigram scores are found to account for the adequacy of the translation, how much information is retained. The longer
-gram scores account for the fluency of the translation, or to what extent it reads like "good English".
The modification made to precision does not solve the problem of short translations, which can produce very high precision scores, even using modified precision. An example of a candidate translation for the same references as above might be:
In this example, the modified unigram precision would be,

as the word 'the' and the word 'cat' appear once each in the candidate, and the total number of words is two. The modified bigram precision would be
as the bigram, "the cat" appears once in the candidate. It has been pointed out that precision is usually twinned with recall to overcome this problem , as the unigram recall of this example would be
or
. The problem being that as there are multiple reference translations, a bad translation could easily have an inflated recall, such as a translation which consisted of all the words in each of the references.
In order to produce a score for the whole corpus the modified precision scores for the segments are combined, using the geometric mean
multiplied by a brevity penalty to prevent very short candidates from receiving too high a score. Let
be the total length of the reference corpus, and
the total length of the translation corpus. If
, the brevity penalty applies, defined to be
. (In the case of multiple reference sentences,
is taken to be the sum of the lengths of the sentences whose lengths are closest to the lengths of the candidate sentences. However, in the version of the metric used by NIST
evaluations prior to 2009, the shortest reference sentence had been used instead.)
It has been argued that although BLEU has significant advantages, there is no guarantee that an increase in BLEU score is an indicator of improved translation quality. Nevertheless, they highlight two instances where BLEU seriously underperformed. These were the 2005 NIST evaluations where a number of different machine translation systems were tested, and their study of the SYSTRAN
engine versus two engines using statistical machine translation
(SMT) techniques.
In the 2005 NIST MT evaluation, it is reported that the scores generated by BLEU failed to correspond to the scores produced in the human evaluations. The system which was ranked highest by the human judges was only ranked 6th by BLEU. In their study, they compared SMT systems with SYSTRAN, a knowledge based system. The scores from BLEU for SYSTRAN were substantially worse than the scores given to SYSTRAN by the human judges. They note that the SMT systems were trained using BLEU minimum error rate training, and point out that this could be one of the reasons behind the
difference. They conclude by recommending that BLEU be used in a more restricted manner, for comparing the results from two similar systems, and for tracking "broad, incremental changes to a single system".
Machine translation
Machine translation, sometimes referred to by the abbreviation MT is a sub-field of computational linguistics that investigates the use of computer software to translate text or speech from one natural language to another.On a basic...
from one natural language
Natural language
In the philosophy of language, a natural language is any language which arises in an unpremeditated fashion as the result of the innate facility for language possessed by the human intellect. A natural language is typically used for communication, and may be spoken, signed, or written...
to another. Quality is considered to be the correspondence between a machine's output and that of a human: "the closer a machine translation is to a professional human translation, the better it is". BLEU was one of the first metrics
Metric (mathematics)
In mathematics, a metric or distance function is a function which defines a distance between elements of a set. A set with a metric is called a metric space. A metric induces a topology on a set but not all topologies can be generated by a metric...
to achieve a high correlation
Correlation
In statistics, dependence refers to any statistical relationship between two random variables or two sets of data. Correlation refers to any of a broad class of statistical relationships involving dependence....
with human judgements of quality, and remains one of the most popular.
Scores are calculated for individual translated segments—generally sentences—by comparing them with a set of good quality reference translations. Those scores are then averaged over the whole corpus
Text corpus
In linguistics, a corpus or text corpus is a large and structured set of texts...
to reach an estimate of the translation's overall quality. Intelligibility or grammatical correctness are not taken into account.
BLEU is designed to approximate human judgement at a corpus level, and performs badly if used to evaluate the quality of individual sentences.
BLEU’s output is always a number between 0 and 1. This value indicates how similar the candidate and reference texts are, with values closer to 1 representing more similar texts.
Algorithm
BLEU uses a modified form of precision to compare a candidate translation against multiple reference translations. The metric modifies simple precision since machine translation systems have been known to generate more words than appear in a reference text. This is illustrated in the following example from Papineni et al. (2002),Candidate | the | the | the | the | the | the | the |
Reference 1 | the | cat | is | on | the | mat | |
Reference 2 | there | is | a | cat | on | the | mat |
Of the seven words in the candidate translation, all of them appear in the reference translations. Thus the candidate text is given a unigram precision of,

where


The modification that BLEU makes is fairly straightforward. For each word in the candidate translation, the algorithm takes its maximum total count,


For the candidate translation, the count






This sum is then divided by the total number of words in the candidate translation. In the above example, the modified unigram precision score would be:

The above method is used to calculate scores for a range of n-gram lengths. The length which has the "highest correlation with monolingual human judgements" was found to be four. The unigram scores are found to account for the adequacy of the translation, how much information is retained. The longer

The modification made to precision does not solve the problem of short translations, which can produce very high precision scores, even using modified precision. An example of a candidate translation for the same references as above might be:
- the cat
In this example, the modified unigram precision would be,

as the word 'the' and the word 'cat' appear once each in the candidate, and the total number of words is two. The modified bigram precision would be



In order to produce a score for the whole corpus the modified precision scores for the segments are combined, using the geometric mean
Geometric mean
The geometric mean, in mathematics, is a type of mean or average, which indicates the central tendency or typical value of a set of numbers. It is similar to the arithmetic mean, except that the numbers are multiplied and then the nth root of the resulting product is taken.For instance, the...
multiplied by a brevity penalty to prevent very short candidates from receiving too high a score. Let





NIST (metric)
NIST is a method for evaluating the quality of text which has been translated using machine translation. Its name comes from the US National Institute of Standards and Technology....
evaluations prior to 2009, the shortest reference sentence had been used instead.)
Performance
BLEU has frequently been reported as correlating well with human judgement, and remains a benchmark for the assessment of any new evaluation metric. There are however a number of criticisms that have been voiced. It has been noted that although in principle capable of evaluating translations of any language, BLEU cannot in its present form deal with languages lacking word boundaries.It has been argued that although BLEU has significant advantages, there is no guarantee that an increase in BLEU score is an indicator of improved translation quality. Nevertheless, they highlight two instances where BLEU seriously underperformed. These were the 2005 NIST evaluations where a number of different machine translation systems were tested, and their study of the SYSTRAN
SYSTRAN
SYSTRAN, founded by Dr. Peter Toma in 1968, is one of the oldest machine translation companies. SYSTRAN has done extensive work for the United States Department of Defense and the European Commission....
engine versus two engines using statistical machine translation
Statistical machine translation
Statistical machine translation is a machine translation paradigm where translations are generated on the basis of statistical models whose parameters are derived from the analysis of bilingual text corpora...
(SMT) techniques.
In the 2005 NIST MT evaluation, it is reported that the scores generated by BLEU failed to correspond to the scores produced in the human evaluations. The system which was ranked highest by the human judges was only ranked 6th by BLEU. In their study, they compared SMT systems with SYSTRAN, a knowledge based system. The scores from BLEU for SYSTRAN were substantially worse than the scores given to SYSTRAN by the human judges. They note that the SMT systems were trained using BLEU minimum error rate training, and point out that this could be one of the reasons behind the
difference. They conclude by recommending that BLEU be used in a more restricted manner, for comparing the results from two similar systems, and for tracking "broad, incremental changes to a single system".
See also
- F-MeasureF1 ScoreIn statistics, the F1 score is a measure of a test's accuracy. It considers both the precision p and the recall r of the test to compute the score: p is the number of correct results divided by the number of all returned results and r is the number of correct results divided by the number of...
- NIST (metric)NIST (metric)NIST is a method for evaluating the quality of text which has been translated using machine translation. Its name comes from the US National Institute of Standards and Technology....
- METEORMETEORMETEOR is a metric for the evaluation of machine translation output. The metric is based on the harmonic mean of unigram precision and recall, with recall weighted higher than precision...
- ROUGE (metric)ROUGE (metric)ROUGE, or Recall-Oriented Understudy for Gisting Evaluation, is a set of metrics and a software package used for evaluating automatic summarization and machine translation software in natural language processing...
- Word Error Rate (WER)Word error rateWord error rate is a common metric of the performance of a speech recognition or machine translation system.The general difficulty of measuring performance lies in the fact that the recognized word sequence can have a different length from the reference word sequence...
- Noun-Phrase Chunking