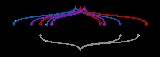
Bloom filter
Encyclopedia
A Bloom filter, conceived by Burton Howard Bloom in 1970, is a space-efficient probabilistic data structure
that is used to test whether an element is a member of a set
. False positives
are possible, but false negative
s are not; i.e. a query returns either "inside set (may be wrong)" or "definitely not in set". Elements can be added to the set, but not removed (though this can be addressed with a counting filter). The more elements that are added to the set, the larger the probability of false positives.
An empty Bloom filter is a bit array of m bits, all set to 0. There must also be k different hash function
s defined, each of which maps
or hashes some set element to one of the m array positions with a uniform random distribution.
To add an element, feed it to each of the k hash functions to get k array positions. Set the bits at all these positions to 1.
To query for an element (test whether it is in the set), feed it to each of the k hash functions to get k array positions. If any of the bits at these positions are 0, the element is not in the set – if it were, then all the bits would have been set to 1 when it was inserted. If all are 1, then either the element is in the set, or the bits have been set to 1 during the insertion of other elements.
The requirement of designing k different independent hash functions can be prohibitive for large k. For a good hash function
with a wide output, there should be little if any correlation between different bit-fields of such a hash, so this type of hash can be used to generate multiple "different" hash functions by slicing its output into multiple bit fields. Alternatively, one can pass k different initial values (such as 0, 1, ..., k − 1) to a hash function that takes an initial value; or add (or append) these values to the key. For larger m and/or k, independence among the hash functions can be relaxed with negligible increase in false positive rate . Specifically, show the effectiveness of deriving the k indices using enhanced double hashing or triple hashing, variants of double hashing
that are effectively simple random number generators seeded with the two or three hash values.
Removing an element from this simple Bloom filter is impossible because false negatives are not permitted. An element maps to k bits, and although setting any one of those k bits to zero suffices to remove the element, it also results in removing any other elements that happen to map onto that bit. Since there is no way of determining whether any other elements have been added that affect the bits for an element to be removed, clearing any of the bits would introduce the possibility for false negatives.
One-time removal of an element from a Bloom filter can be simulated by having a second Bloom filter that contains items that have been removed. However, false positives in the second filter become false negatives in the composite filter, which are not permitted. In this approach re-adding a previously removed item is not possible, as one would have to remove it from the "removed" filter.
However, it is often the case that all the keys are available but are expensive to enumerate (for example, requiring many disk reads). When the false positive rate gets too high, the filter can be regenerated; this should be a relatively rare event.
s, trie
s, hash table
s, or simple arrays or linked list
s of the entries. Most of these require storing at least the data items themselves, which can require anywhere from a small number of bits, for small integers, to an arbitrary number of bits, such as for strings (tries are an exception, since they can share storage between elements with equal prefixes). Linked structures incur an additional linear space overhead for pointers. A Bloom filter with 1% error and an optimal value of k, in contrast, requires only about 9.6 bits per element — regardless of the size of the elements. This advantage comes partly from its compactness, inherited from arrays, and partly from its probabilistic nature. If a 1% false-positive rate seems too high, adding about 4.8 bits per element decreases it by ten times.
However, if the number of potential values is small and many of them can be in the set, the Bloom filter is easily surpassed by the deterministic bit array, which requires only one bit for each potential element. Note also that hash tables gain a space and time advantage if they begin ignoring collisions and store only whether each bucket contains an entry; in this case, they have effectively become Bloom filters with k = 1.
Bloom filters also have the unusual property that the time needed either to add items or to check whether an item is in the set is a fixed constant, O(k), completely independent of the number of items already in the set. No other constant-space set data structure has this property, but the average access time of sparse hash table
s can make them faster in practice than some Bloom filters. In a hardware implementation, however, the Bloom filter shines because its k lookups are independent and can be parallelized.
To understand its space efficiency, it is instructive to compare the general Bloom filter with its special case when k = 1. If k = 1, then in order to keep the false positive rate sufficiently low, a small fraction of bits should be set, which means the array must be very large and contain long runs of zeros. The information content
of the array relative to its size is low. The generalized Bloom filter (k greater than 1) allows many more bits to be set while still maintaining a low false positive rate; if the parameters (k and m) are chosen well, about half of the bits will be set, and these will be apparently random, minimizing redundancy and maximizing information content.
Assume that a hash function
selects each array position with equal probability. If m is the number of bits in the array, the probability that a certain bit is not set to one by a certain hash function during the insertion of an element is then

The probability that it is not set by any of the hash functions is

If we have inserted n elements, the probability that a certain bit is still 0 is

the probability that it is 1 is therefore

Now test membership of an element that is not in the set. Each of the k array positions computed by the hash functions is 1 with a probability as above. The probability of all of them being 1, which would cause the algorithm
to erroneously claim that the element is in the set, is often given as

This is not strictly correct as it assumes independence for the probabilities of each bit being set. However, assuming it is a close approximation we have that the probability of false positives decreases as m (the number of bits in the array) increases, and increases as n (the number of inserted elements) increases. For a given m and n, the value of k (the number of hash functions) that minimizes the probability is

which gives the false positive probability of

The required number of bits m, given n (the number of inserted elements) and a desired false positive probability p (and assuming the optimal value of k is used) can be computed by substituting the optimal value of k in the probability expression above:
which can be simplified to:
This results in:
This means that in order to maintain a fixed false positive probability, the length of a Bloom filter must grow linearly with the number of elements being filtered. While the above formula is asymptotic (i.e. applicable as m, n → ∞), the agreement with finite values of m,n is also quite good; the false positive probability for a finite bloom filter with m bits, n elements, and k hash functions is at most

So we can use the asymptotic formula if we pay a penalty for at most half an extra element and at most one fewer bit .
uses Bloom filters to reduce the disk lookups for non-existent rows or columns. Avoiding costly disk lookups considerably increases the performance of a database query operation.
The Squid Web
Proxy Cache
uses Bloom filters for cache digests.
The Venti
archival storage system uses Bloom filters to detect previously-stored data.
The SPIN model checker
uses Bloom filters to track the reachable state space for large verification problems.
The Google Chrome
web browser
uses Bloom filters to speed up its Safe Browsing service.
bits of space per inserted key, where
is the false positive rate of the Bloom filter. However, the space that is strictly necessary for any data structure playing the same role as a Bloom filter is only
per key . Hence Bloom filters use 44% more space than a hypothetical equivalent optimal data structure. The number of hash functions used to achieve a given false positive rate
is proportional to
which is not optimal as it has been proved that an optimal data structure would need only a constant number of hash functions independent of the false positive rate.
describe a probabilistic structure based on hash table
s, hash compaction, which identify as significantly more accurate than a Bloom filter when each is configured optimally. Dillinger and Manolios, however, point out that the reasonable accuracy of any given Bloom filter over a wide range of numbers of additions makes it attractive for probabilistic enumeration of state spaces of unknown size. Hash compaction is, therefore, attractive when the number of additions can be predicted accurately; however, despite being very fast in software, hash compaction is poorly-suited for hardware because of worst-case linear access time.
have studied some variants of Bloom filters that are either faster or use less space than classic Bloom filters. The basic idea of the fast variant is to locate the k hash values associated with each key into one or two blocks having the same size as processor's memory cache blocks (usually 64 bytes). This will presumably improve performance by reducing the number of potential memory cache misses. The proposed variants have however the drawback of using about 32% more space than classic Bloom filters.
The space efficient variant relies on using a single hash function that generates for each key a value in the range
where
is the requested false positive rate. The sequence of values is then sorted and compressed using Golomb coding
(or some other compression technique) to occupy a space close to
bits. To query the Bloom filter for a given key, it will suffice to check if its corresponding value is stored in the Bloom filter. Decompressing the whole Bloom filter for each query would make this variant totally unusable. To overcome this problem the sequence of values is divided into small blocks of equal size that are compressed separately. At query time only half a block will need to be decompressed on average. Because of decompression overhead, this variant may be slower than classic Bloom filters but this may be compensated by the fact that a single hash function need to be computed.
Another alternative to classic Bloom filter is the one based on space efficient variants of cuckoo hashing
. In this case once the hash table is constructed, the keys stored in the hash table are replaced with short signatures of the keys. Those signatures are strings of bits computed using a hash function applied on the keys.
The insert operation is extended to increment the value of the buckets and the lookup operation checks that each of the required buckets is non-zero. The delete operation, obviously, then consists of decrementing the value of each of the respective buckets.
Arithmetic overflow
of the buckets is a problem and the buckets should be sufficiently large to make this case rare. If it does occur then the increment and decrement operations must leave the bucket set to the maximum possible value in order to retain the properties of a Bloom filter.
The size of counters is usually 3 or 4 bits. Hence counting Bloom filters use 3 to 4 times more space than static Bloom filters. In theory, an optimal data structure equivalent to a counting Bloom filter should not use more space than a static Bloom filter.
Another issue with counting filters is limited scalability. Because the counting Bloom filter table cannot be expanded, the maximal number of keys to be stored simultaneously in the filter must be known in advance. Once the designed capacity of the table is exceeded the false positive rate will grow rapidly as more keys are inserted.
introduced a data structure based on d-left hashing that is functionally equivalent but uses approximately half as much space as counting Bloom filters. The scalability issue does not occur in this data structure. Once the designed capacity is exceeded, the keys could be reinserted in a new hash table of double size.
The space efficient variant by could also be used to implement counting filters by supporting insertions and deletions.
as in . Counting Bloom filters can be used to approximate the number of differences between two sets and this approach is described in .
. Like Bloom filters, these structures achieve a small space overhead by accepting a small probability of false positives. In the case of "Bloomier filters", a false positive is defined as returning a result when the key is not in the map. The map will never return the wrong value for a key that is in the map.
The simplest Bloomier filter is near-optimal and fairly simple to describe. Suppose initially that the only possible values are 0 and 1. We create a pair of Bloom filters A0 and B0 which contain, respectively, all keys mapping to 0 and all keys mapping to 1. Then, to determine which value a given key maps to, we look it up in both filters. If it is in neither, then the key is not in the map. If the key is in A0 but not B0, then it does not map to 1, and has a high probability of mapping to 0. Conversely, if the key is in B0 but not A0, then it does not map to 0 and has a high probability of mapping to 1.
A problem arises, however, when both filters claim to contain the key. We never insert a key into both, so one or both of the filters is lying (producing a false positive), but we don't know which. To determine this, we have another, smaller pair of filters A1 and B1. A1 contains keys that map to 0 and which are false positives in B0; B1 contains keys that map to 1 and which are false positives in A0. But whenever A0 and B0 both produce positives, at most one of these cases must occur, and so we simply have to determine which if any of the two filters A1 and B1 contains the key, another instance of our original problem.
It may so happen again that both filters produce a positive; we apply the same idea recursively to solve this problem. Because each pair of filters only contains keys that are in the map and produced false positives on all previous filter pairs, the number of keys is extremely likely to quickly drop to a very small quantity that can be easily stored in an ordinary deterministic map, such as a pair of small arrays with linear search. Moreover, the average total search time is a constant, because almost all queries will be resolved by the first pair, almost all remaining queries by the second pair, and so on. The total space required is independent of n, and is almost entirely occupied by the first filter pair.
Now that we have the structure and a search algorithm, we also need to know how to insert new key/value pairs. The program must not attempt to insert the same key with both values. If the value is 0, insert the key into A0 and then test if the key is in B0. If so, this is a false positive for B0, and the key must also be inserted into A1 recursively in the same manner. If we reach the last level, we simply insert it. When the value is 1, the operation is similar but with A and B reversed.
Now that we can map a key to the value 0 or 1, how does this help us map to general values? This is simple. We create a single such Bloomier filter for each bit of the result. If the values are large, we can instead map keys to hash values that can be used to retrieve the actual values. The space required for a Bloomier filter with n-bit values is typically slightly more than the space for 2n Bloom filters.
A very simple way to implement Bloomier filters is by means of minimal perfect hashing. A minimal perfect hash function h is first generated for the set of n keys. Then an array is filled with n pairs (signature,value) associated with each key at the positions given by function h when applied on each key. The signature of a key is a string of r bits computed by applying a hash function g of range
on the key. The value of r is chosen such that
, where
is the requested false positive rate. To query for a given key, hash function h is first applied on the key. This will give a position into the array from which we retrieve a pair (signature,value). Then we compute the signature of the key using function g. If the computed signature is the same as retrieved signature we return the retrieved value. The probability of false positive is
.
Another alternative to implement static bloomier and bloom filters based on matrix solving has been simultaneously proposed in , and . The space usage of this method is optimal as it needs only
bits per key for a bloom filter. However time to generate the bloom or bloomier filter can be very high. The generation time can be reduced to a reasonable value at the price of a small increase in space usage.
Dynamic Bloomier filters have been studied by . They proved that any dynamic Bloomier filter needs at least around
bits per key where l is the length of the key. A simple dynamic version of Bloomier filters can be implemented using two dynamic data structures. Let the two data structures be noted S1 and S2. S1 will store keys with their associated data while S2 will only store signatures of keys with their associated data. Those signatures are simply hash values of keys in the range
where n is the maximal number of keys to be stored in the Bloomier filter and
is the requested false positive rate. To insert a key in the Bloomier filter, its hash value is first computed. Then the algorithm checks if a key with the same hash value already exists in S2. If this is not the case, the hash value is inserted in S2 along with data associated with the key. If the same hash value already exists in S2 then the key is inserted into S1 along with its associated data. The deletion is symmetric: if the key already exists in S1 it will be deleted from there, otherwise the hash value associated with the key is deleted from S2. An issue with this algorithm is on how to store efficiently S1 and S2. For S1 any hash algorithm can be used. To store S2 golomb coding
could be applied to compress signatures to use a space close to
per key.
-based generalization of Bloom filters. A compact approximator associates to each key an element of a lattice (the standard Bloom filters being the case of the Boolean two-element lattice). Instead of a bit array, they have an array of lattice elements. When adding a new association between a key and an element of the lattice, they maximize the current content of the k array locations associated to the key with the lattice element. When reading the value associated to a key, they minimize the values found in the k locations associated to the key. The resulting value approximates from above the original value.
Lets take a small network shown on the graph below as an example. Say we are searching for a service A whose id hashes to bits 0,1, and 3 (pattern 11010). Let n1 node to be the starting point. First, we check whether service A is offered by n1 by checking its local filter. Since the patterns don't match, we check the attenuated bloom filter in order to determine which node should be the next hop. We see that n2 doesn't offer service A but lies on the path to nodes that do. Hence, we move to n2 and repeat the same procedure. We quickly find that n3 offers the service, and hence the destination is located.
By using attenuated Bloom filters consisting of multiple layers, services at more than one hop distance can be discovered while avoiding saturation of the Bloom filter by attenuating (shifting out) bits set by sources further away.
Data structure
In computer science, a data structure is a particular way of storing and organizing data in a computer so that it can be used efficiently.Different kinds of data structures are suited to different kinds of applications, and some are highly specialized to specific tasks...
that is used to test whether an element is a member of a set
Set (computer science)
In computer science, a set is an abstract data structure that can store certain values, without any particular order, and no repeated values. It is a computer implementation of the mathematical concept of a finite set...
. False positives
Type I and type II errors
In statistical test theory the notion of statistical error is an integral part of hypothesis testing. The test requires an unambiguous statement of a null hypothesis, which usually corresponds to a default "state of nature", for example "this person is healthy", "this accused is not guilty" or...
are possible, but false negative
Type I and type II errors
In statistical test theory the notion of statistical error is an integral part of hypothesis testing. The test requires an unambiguous statement of a null hypothesis, which usually corresponds to a default "state of nature", for example "this person is healthy", "this accused is not guilty" or...
s are not; i.e. a query returns either "inside set (may be wrong)" or "definitely not in set". Elements can be added to the set, but not removed (though this can be addressed with a counting filter). The more elements that are added to the set, the larger the probability of false positives.
Algorithm description
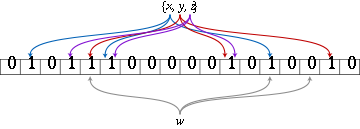
Hash function
A hash function is any algorithm or subroutine that maps large data sets to smaller data sets, called keys. For example, a single integer can serve as an index to an array...
s defined, each of which maps
Map (mathematics)
In most of mathematics and in some related technical fields, the term mapping, usually shortened to map, is either a synonym for function, or denotes a particular kind of function which is important in that branch, or denotes something conceptually similar to a function.In graph theory, a map is a...
or hashes some set element to one of the m array positions with a uniform random distribution.
To add an element, feed it to each of the k hash functions to get k array positions. Set the bits at all these positions to 1.
To query for an element (test whether it is in the set), feed it to each of the k hash functions to get k array positions. If any of the bits at these positions are 0, the element is not in the set – if it were, then all the bits would have been set to 1 when it was inserted. If all are 1, then either the element is in the set, or the bits have been set to 1 during the insertion of other elements.
The requirement of designing k different independent hash functions can be prohibitive for large k. For a good hash function
Hash function
A hash function is any algorithm or subroutine that maps large data sets to smaller data sets, called keys. For example, a single integer can serve as an index to an array...
with a wide output, there should be little if any correlation between different bit-fields of such a hash, so this type of hash can be used to generate multiple "different" hash functions by slicing its output into multiple bit fields. Alternatively, one can pass k different initial values (such as 0, 1, ..., k − 1) to a hash function that takes an initial value; or add (or append) these values to the key. For larger m and/or k, independence among the hash functions can be relaxed with negligible increase in false positive rate . Specifically, show the effectiveness of deriving the k indices using enhanced double hashing or triple hashing, variants of double hashing
Double hashing
Double hashing is a computer programming technique used in hash tables to resolve hash collisions, cases when two different values to be searched for produce the same hash key...
that are effectively simple random number generators seeded with the two or three hash values.
Removing an element from this simple Bloom filter is impossible because false negatives are not permitted. An element maps to k bits, and although setting any one of those k bits to zero suffices to remove the element, it also results in removing any other elements that happen to map onto that bit. Since there is no way of determining whether any other elements have been added that affect the bits for an element to be removed, clearing any of the bits would introduce the possibility for false negatives.
One-time removal of an element from a Bloom filter can be simulated by having a second Bloom filter that contains items that have been removed. However, false positives in the second filter become false negatives in the composite filter, which are not permitted. In this approach re-adding a previously removed item is not possible, as one would have to remove it from the "removed" filter.
However, it is often the case that all the keys are available but are expensive to enumerate (for example, requiring many disk reads). When the false positive rate gets too high, the filter can be regenerated; this should be a relatively rare event.
Space and time advantages
While risking false positives, Bloom filters have a strong space advantage over other data structures for representing sets, such as self-balancing binary search treeSelf-balancing binary search tree
In computer science, a self-balancing binary search tree is any node based binary search tree that automatically keeps its height small in the face of arbitrary item insertions and deletions....
s, trie
Trie
In computer science, a trie, or prefix tree, is an ordered tree data structure that is used to store an associative array where the keys are usually strings. Unlike a binary search tree, no node in the tree stores the key associated with that node; instead, its position in the tree defines the...
s, hash table
Hash table
In computer science, a hash table or hash map is a data structure that uses a hash function to map identifying values, known as keys , to their associated values . Thus, a hash table implements an associative array...
s, or simple arrays or linked list
Linked list
In computer science, a linked list is a data structure consisting of a group of nodes which together represent a sequence. Under the simplest form, each node is composed of a datum and a reference to the next node in the sequence; more complex variants add additional links...
s of the entries. Most of these require storing at least the data items themselves, which can require anywhere from a small number of bits, for small integers, to an arbitrary number of bits, such as for strings (tries are an exception, since they can share storage between elements with equal prefixes). Linked structures incur an additional linear space overhead for pointers. A Bloom filter with 1% error and an optimal value of k, in contrast, requires only about 9.6 bits per element — regardless of the size of the elements. This advantage comes partly from its compactness, inherited from arrays, and partly from its probabilistic nature. If a 1% false-positive rate seems too high, adding about 4.8 bits per element decreases it by ten times.
However, if the number of potential values is small and many of them can be in the set, the Bloom filter is easily surpassed by the deterministic bit array, which requires only one bit for each potential element. Note also that hash tables gain a space and time advantage if they begin ignoring collisions and store only whether each bucket contains an entry; in this case, they have effectively become Bloom filters with k = 1.
Bloom filters also have the unusual property that the time needed either to add items or to check whether an item is in the set is a fixed constant, O(k), completely independent of the number of items already in the set. No other constant-space set data structure has this property, but the average access time of sparse hash table
Hash table
In computer science, a hash table or hash map is a data structure that uses a hash function to map identifying values, known as keys , to their associated values . Thus, a hash table implements an associative array...
s can make them faster in practice than some Bloom filters. In a hardware implementation, however, the Bloom filter shines because its k lookups are independent and can be parallelized.
To understand its space efficiency, it is instructive to compare the general Bloom filter with its special case when k = 1. If k = 1, then in order to keep the false positive rate sufficiently low, a small fraction of bits should be set, which means the array must be very large and contain long runs of zeros. The information content
Information content
The term information content is used to refer the meaning of information as opposed to the form or carrier of the information. For example, the meaning that is conveyed in an expression or document, which can be distinguished from the sounds or symbols or codes and carrier that physically form the...
of the array relative to its size is low. The generalized Bloom filter (k greater than 1) allows many more bits to be set while still maintaining a low false positive rate; if the parameters (k and m) are chosen well, about half of the bits will be set, and these will be apparently random, minimizing redundancy and maximizing information content.
Probability of false positives
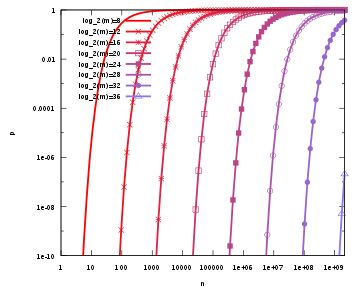
Hash function
A hash function is any algorithm or subroutine that maps large data sets to smaller data sets, called keys. For example, a single integer can serve as an index to an array...
selects each array position with equal probability. If m is the number of bits in the array, the probability that a certain bit is not set to one by a certain hash function during the insertion of an element is then

The probability that it is not set by any of the hash functions is

If we have inserted n elements, the probability that a certain bit is still 0 is

the probability that it is 1 is therefore

Now test membership of an element that is not in the set. Each of the k array positions computed by the hash functions is 1 with a probability as above. The probability of all of them being 1, which would cause the algorithm
Algorithm
In mathematics and computer science, an algorithm is an effective method expressed as a finite list of well-defined instructions for calculating a function. Algorithms are used for calculation, data processing, and automated reasoning...
to erroneously claim that the element is in the set, is often given as

This is not strictly correct as it assumes independence for the probabilities of each bit being set. However, assuming it is a close approximation we have that the probability of false positives decreases as m (the number of bits in the array) increases, and increases as n (the number of inserted elements) increases. For a given m and n, the value of k (the number of hash functions) that minimizes the probability is

which gives the false positive probability of

The required number of bits m, given n (the number of inserted elements) and a desired false positive probability p (and assuming the optimal value of k is used) can be computed by substituting the optimal value of k in the probability expression above:

which can be simplified to:

This results in:

This means that in order to maintain a fixed false positive probability, the length of a Bloom filter must grow linearly with the number of elements being filtered. While the above formula is asymptotic (i.e. applicable as m, n → ∞), the agreement with finite values of m,n is also quite good; the false positive probability for a finite bloom filter with m bits, n elements, and k hash functions is at most

So we can use the asymptotic formula if we pay a penalty for at most half an extra element and at most one fewer bit .
Interesting properties
- Unlike sets based on hash tableHash tableIn computer science, a hash table or hash map is a data structure that uses a hash function to map identifying values, known as keys , to their associated values . Thus, a hash table implements an associative array...
s, any Bloom filter can represent the entire universeUniverse (mathematics)In mathematics, and particularly in set theory and the foundations of mathematics, a universe is a class that contains all the entities one wishes to consider in a given situation...
of elements. In this case, all bits are 1. Another consequence of this property is that add never fails due to the data structure "filling up." However, the false positive rate increases steadily as elements are added until all bits in the filter are set to 1, so a negative value is never returned. At this point, the Bloom filter completely ceases to differentiate between differing inputs, and is functionally useless.
- UnionUnion (set theory)In set theory, the union of a collection of sets is the set of all distinct elements in the collection. The union of a collection of sets S_1, S_2, S_3, \dots , S_n\,\! gives a set S_1 \cup S_2 \cup S_3 \cup \dots \cup S_n.- Definition :...
and intersectionIntersection (set theory)In mathematics, the intersection of two sets A and B is the set that contains all elements of A that also belong to B , but no other elements....
of Bloom filters with the same size and set of hash functions can be implemented with bitwiseBitwise operationA bitwise operation operates on one or more bit patterns or binary numerals at the level of their individual bits. This is used directly at the digital hardware level as well as in microcode, machine code and certain kinds of high level languages...
OR and AND operations, respectively. The union operation on Bloom filters is lossless in the sense that the resulting Bloom filter is the same as the Bloom filter created from scratch using the union of the two sets. The intersect operation satisfies a weaker property: the false positive probability in the resulting Bloom filter is at most the false-positive probability in one of the constituent Bloom filters, but may be larger than the false positive probability in the Bloom filter created from scratch using the intersection of the two sets.
Examples
Google BigTableBigTable
BigTable is a compressed, high performance, and proprietary database system built on Google File System , Chubby Lock Service, SSTable and a few other Google technologies; it is currently not distributed nor is it used outside of Google, although Google offers access to it as part of their Google...
uses Bloom filters to reduce the disk lookups for non-existent rows or columns. Avoiding costly disk lookups considerably increases the performance of a database query operation.
The Squid Web
World Wide Web
The World Wide Web is a system of interlinked hypertext documents accessed via the Internet...
Proxy Cache
Cache
In computer engineering, a cache is a component that transparently stores data so that future requests for that data can be served faster. The data that is stored within a cache might be values that have been computed earlier or duplicates of original values that are stored elsewhere...
uses Bloom filters for cache digests.
The Venti
Venti
Venti is a network storage system that permanently stores data blocks. A 160-bit SHA-1 hash of the data acts as the address of the data...
archival storage system uses Bloom filters to detect previously-stored data.
The SPIN model checker
SPIN model checker
SPIN is a general tool for verifying the correctness of distributed software models in a rigorous and mostly automated fashion. It was written by Gerard J. Holzmann and others in the original Unix group of the Computing Sciences Research Center at Bell Labs, beginning in 1980...
uses Bloom filters to track the reachable state space for large verification problems.
The Google Chrome
Google Chrome
Google Chrome is a web browser developed by Google that uses the WebKit layout engine. It was first released as a beta version for Microsoft Windows on September 2, 2008, and the public stable release was on December 11, 2008. The name is derived from the graphical user interface frame, or...
web browser
Web browser
A web browser is a software application for retrieving, presenting, and traversing information resources on the World Wide Web. An information resource is identified by a Uniform Resource Identifier and may be a web page, image, video, or other piece of content...
uses Bloom filters to speed up its Safe Browsing service.
Alternatives
Classic Bloom filters use




describe a probabilistic structure based on hash table
Hash table
In computer science, a hash table or hash map is a data structure that uses a hash function to map identifying values, known as keys , to their associated values . Thus, a hash table implements an associative array...
s, hash compaction, which identify as significantly more accurate than a Bloom filter when each is configured optimally. Dillinger and Manolios, however, point out that the reasonable accuracy of any given Bloom filter over a wide range of numbers of additions makes it attractive for probabilistic enumeration of state spaces of unknown size. Hash compaction is, therefore, attractive when the number of additions can be predicted accurately; however, despite being very fast in software, hash compaction is poorly-suited for hardware because of worst-case linear access time.
have studied some variants of Bloom filters that are either faster or use less space than classic Bloom filters. The basic idea of the fast variant is to locate the k hash values associated with each key into one or two blocks having the same size as processor's memory cache blocks (usually 64 bytes). This will presumably improve performance by reducing the number of potential memory cache misses. The proposed variants have however the drawback of using about 32% more space than classic Bloom filters.
The space efficient variant relies on using a single hash function that generates for each key a value in the range


Golomb coding
Golomb coding is a lossless data compression method using a family of data compression codes invented by Solomon W. Golomb in the 1960s. Alphabets following a geometric distribution will have a Golomb code as an optimal prefix code, making Golomb coding highly suitable for situations in which the...
(or some other compression technique) to occupy a space close to

Another alternative to classic Bloom filter is the one based on space efficient variants of cuckoo hashing
Cuckoo hashing
Cuckoo hashing is a scheme in computer programming for resolving hash collisions of values of hash functions in a table. Cuckoo hashing was first described by Rasmus Pagh and Flemming Friche Rodler in 2001...
. In this case once the hash table is constructed, the keys stored in the hash table are replaced with short signatures of the keys. Those signatures are strings of bits computed using a hash function applied on the keys.
Counting filters
Counting filters provide a way to implement a delete operation on a Bloom filter without recreating the filter afresh. In a counting filter the array positions (buckets) are extended from being a single bit, to an n-bit counter. In fact, regular Bloom filters can be considered as counting filters with a bucket size of one bit. Counting filters were introduced by .The insert operation is extended to increment the value of the buckets and the lookup operation checks that each of the required buckets is non-zero. The delete operation, obviously, then consists of decrementing the value of each of the respective buckets.
Arithmetic overflow
Arithmetic overflow
The term arithmetic overflow or simply overflow has the following meanings.# In a computer, the condition that occurs when a calculation produces a result that is greater in magnitude than that which a given register or storage location can store or represent.# In a computer, the amount by which a...
of the buckets is a problem and the buckets should be sufficiently large to make this case rare. If it does occur then the increment and decrement operations must leave the bucket set to the maximum possible value in order to retain the properties of a Bloom filter.
The size of counters is usually 3 or 4 bits. Hence counting Bloom filters use 3 to 4 times more space than static Bloom filters. In theory, an optimal data structure equivalent to a counting Bloom filter should not use more space than a static Bloom filter.
Another issue with counting filters is limited scalability. Because the counting Bloom filter table cannot be expanded, the maximal number of keys to be stored simultaneously in the filter must be known in advance. Once the designed capacity of the table is exceeded the false positive rate will grow rapidly as more keys are inserted.
introduced a data structure based on d-left hashing that is functionally equivalent but uses approximately half as much space as counting Bloom filters. The scalability issue does not occur in this data structure. Once the designed capacity is exceeded, the keys could be reinserted in a new hash table of double size.
The space efficient variant by could also be used to implement counting filters by supporting insertions and deletions.
Data synchronization
Bloom filters can be used for approximate data synchronizationData synchronization
Data synchronization is the process of establishing consistency among data from a source to a target data storage and vice versa and the continuous harmonization of the data over time. It is fundamental to a wide variety of applications, including file synchronization and mobile device...
as in . Counting Bloom filters can be used to approximate the number of differences between two sets and this approach is described in .
Bloomier filters
designed a generalization of Bloom filters that could associate a value with each element that had been inserted, implementing an associative arrayAssociative array
In computer science, an associative array is an abstract data type composed of a collection of pairs, such that each possible key appears at most once in the collection....
. Like Bloom filters, these structures achieve a small space overhead by accepting a small probability of false positives. In the case of "Bloomier filters", a false positive is defined as returning a result when the key is not in the map. The map will never return the wrong value for a key that is in the map.
The simplest Bloomier filter is near-optimal and fairly simple to describe. Suppose initially that the only possible values are 0 and 1. We create a pair of Bloom filters A0 and B0 which contain, respectively, all keys mapping to 0 and all keys mapping to 1. Then, to determine which value a given key maps to, we look it up in both filters. If it is in neither, then the key is not in the map. If the key is in A0 but not B0, then it does not map to 1, and has a high probability of mapping to 0. Conversely, if the key is in B0 but not A0, then it does not map to 0 and has a high probability of mapping to 1.
A problem arises, however, when both filters claim to contain the key. We never insert a key into both, so one or both of the filters is lying (producing a false positive), but we don't know which. To determine this, we have another, smaller pair of filters A1 and B1. A1 contains keys that map to 0 and which are false positives in B0; B1 contains keys that map to 1 and which are false positives in A0. But whenever A0 and B0 both produce positives, at most one of these cases must occur, and so we simply have to determine which if any of the two filters A1 and B1 contains the key, another instance of our original problem.
It may so happen again that both filters produce a positive; we apply the same idea recursively to solve this problem. Because each pair of filters only contains keys that are in the map and produced false positives on all previous filter pairs, the number of keys is extremely likely to quickly drop to a very small quantity that can be easily stored in an ordinary deterministic map, such as a pair of small arrays with linear search. Moreover, the average total search time is a constant, because almost all queries will be resolved by the first pair, almost all remaining queries by the second pair, and so on. The total space required is independent of n, and is almost entirely occupied by the first filter pair.
Now that we have the structure and a search algorithm, we also need to know how to insert new key/value pairs. The program must not attempt to insert the same key with both values. If the value is 0, insert the key into A0 and then test if the key is in B0. If so, this is a false positive for B0, and the key must also be inserted into A1 recursively in the same manner. If we reach the last level, we simply insert it. When the value is 1, the operation is similar but with A and B reversed.
Now that we can map a key to the value 0 or 1, how does this help us map to general values? This is simple. We create a single such Bloomier filter for each bit of the result. If the values are large, we can instead map keys to hash values that can be used to retrieve the actual values. The space required for a Bloomier filter with n-bit values is typically slightly more than the space for 2n Bloom filters.
A very simple way to implement Bloomier filters is by means of minimal perfect hashing. A minimal perfect hash function h is first generated for the set of n keys. Then an array is filled with n pairs (signature,value) associated with each key at the positions given by function h when applied on each key. The signature of a key is a string of r bits computed by applying a hash function g of range




Another alternative to implement static bloomier and bloom filters based on matrix solving has been simultaneously proposed in , and . The space usage of this method is optimal as it needs only

Dynamic Bloomier filters have been studied by . They proved that any dynamic Bloomier filter needs at least around



Golomb coding
Golomb coding is a lossless data compression method using a family of data compression codes invented by Solomon W. Golomb in the 1960s. Alphabets following a geometric distribution will have a Golomb code as an optimal prefix code, making Golomb coding highly suitable for situations in which the...
could be applied to compress signatures to use a space close to

Compact approximators
proposed a latticeLattice (order)
In mathematics, a lattice is a partially ordered set in which any two elements have a unique supremum and an infimum . Lattices can also be characterized as algebraic structures satisfying certain axiomatic identities...
-based generalization of Bloom filters. A compact approximator associates to each key an element of a lattice (the standard Bloom filters being the case of the Boolean two-element lattice). Instead of a bit array, they have an array of lattice elements. When adding a new association between a key and an element of the lattice, they maximize the current content of the k array locations associated to the key with the lattice element. When reading the value associated to a key, they minimize the values found in the k locations associated to the key. The resulting value approximates from above the original value.
Stable Bloom filters
proposed Stable Bloom filters as a variant of Bloom filters for streaming data. The idea is that since there is no way to store the entire history of a stream (which can be infinite), Stable Bloom filters continuously evict stale information to make room for more recent elements. Since stale information is evicted, the Stable Bloom filter introduces false negatives, which do not appear in traditional bloom filters. The authors show that a tight upper bound of false positive rates is guaranteed, and the method is superior to standard bloom filters in terms of false positive rates and time efficiency when a small space and an acceptable false positive rate are given.Scalable Bloom filters
proposed a variant of Bloom filters that can adapt dynamically to the number of elements stored, while assuring a minimum false positive probability. The technique is based on sequences of standard bloom filters with increasing capacity and tighter false positive probabilities, so as to ensure that a maximum false positive probability can be set beforehand, regardless of the number of elements to be inserted.Attenuated Bloom filters
An attenuated bloom filter of depth D can be viewed as an array of D normal bloom filters. In the context of service discovery in a network, each node stores regular and attenuated bloom filters locally. The regular or local bloom filter indicates which services are offered by the node itself. The attenuated filter of level i indicates which services can be found on nodes that are i-hops away from the current node. The i-th value is constructed by taking a union of local bloom filters for nodes i-hops away from the node.Lets take a small network shown on the graph below as an example. Say we are searching for a service A whose id hashes to bits 0,1, and 3 (pattern 11010). Let n1 node to be the starting point. First, we check whether service A is offered by n1 by checking its local filter. Since the patterns don't match, we check the attenuated bloom filter in order to determine which node should be the next hop. We see that n2 doesn't offer service A but lies on the path to nodes that do. Hence, we move to n2 and repeat the same procedure. We quickly find that n3 offers the service, and hence the destination is located.
By using attenuated Bloom filters consisting of multiple layers, services at more than one hop distance can be discovered while avoiding saturation of the Bloom filter by attenuating (shifting out) bits set by sources further away.
External links
- Table of false-positive rates for different configurations from a University of Wisconsin–MadisonUniversity of Wisconsin–MadisonThe University of Wisconsin–Madison is a public research university located in Madison, Wisconsin, United States. Founded in 1848, UW–Madison is the flagship campus of the University of Wisconsin System. It became a land-grant institution in 1866...
website - Bloom Filters and Social Networks with Java applet demo from a Sun MicrosystemsSun MicrosystemsSun Microsystems, Inc. was a company that sold :computers, computer components, :computer software, and :information technology services. Sun was founded on February 24, 1982...
website - Interactive Processing demonstration from ashcan.org
- "More Optimal Bloom Filters," Ely Porat (Nov/2007) Google TechTalk video on YouTubeYouTubeYouTube is a video-sharing website, created by three former PayPal employees in February 2005, on which users can upload, view and share videos....
- "Using Bloom Filters" Detailed Bloom Filter explanation using PerlPerlPerl is a high-level, general-purpose, interpreted, dynamic programming language. Perl was originally developed by Larry Wall in 1987 as a general-purpose Unix scripting language to make report processing easier. Since then, it has undergone many changes and revisions and become widely popular...
Implementations
- Implementation in C from literateprograms.org
- Implementation in C++ and Object Pascal from partow.net
- Implementation in C# from codeplex.com
- Implementation in Erlang from sites.google.com
- Implementation in Haskell from haskell.org
- Implementation in Java from tu-dresden.de
- Implementation in Javascript from la.ma.la
- Implementation in Lisp from lemonodor.com
- Implementation in Perl from cpan.org
- Implementation in PHP from code.google.com
- Implementation in Python, Scalable Bloom Filter from pypi.python.org
- Implementation in Ruby from rubyinside.com
- Implementation in Scala from codecommit.com
- Implementation in Tcl from kocjan.org