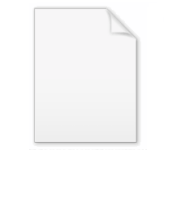
Effect size
Encyclopedia
In statistics
, an effect size is a measure of the strength of the relationship between two variables in a statistical population
, or a sample-based estimate of that quantity. An effect size calculated from data
is a descriptive statistic
that conveys the estimated magnitude of a relationship without making any statement about whether the apparent relationship in the data reflects a true relationship in the population
. In that way, effect sizes complement inferential statistics such as p-values. Among other uses, effect size measures play an important role in meta-analysis
studies that summarize findings from a specific area of research, and in statistical power
analyses.
The concept of effect size appears already in everyday language. For example, a weight loss program may boast that it leads to an average weight loss of 30 pounds. In this case, 30 pounds is an indicator of the claimed effect size. Another example is that a tutoring program may claim that it raises school performance by one letter grade. This grade increase is the claimed effect size of the program. These are both examples of "absolute effect sizes," meaning that they convey the average difference between two groups without any discussion of the variability
within the groups. For example, if the weight loss program results in an average loss of 30 pounds, it is possible that every participant loses exactly 30 pounds, or half the participants lose 60 pounds and half lose no weight at all.
Reporting effect sizes is considered good practice when presenting empirical research findings in many fields. The reporting of effect sizes facilitates the interpretation of the substantive, as opposed to the statistical, significance of a research result.
Effect sizes are particularly prominent in social and medical research. Relative and absolute measures of effect size convey different information, and can be used complementarily. A prominent task force in the psychology research community expressed the following recommendation:
, or to a parameter of a hypothetical statistical population. Conventions for distinguishing sample from population effect sizes follow standard statistical practices — one common approach is to use Greek letters like ρ to denote population parameters and Latin letters like r to denote the corresponding statistic; alternatively, a "hat" can be placed over the population parameter to denote the statistic, e.g. with ρ̂ being the estimate of the parameter ρ.
As in any statistical setting, effect sizes are estimated with error, and may be biased unless the effect size estimator that is used is appropriate for the manner in which the data were sampled
and the manner in which the measurements were made. An example of this is publication bias
, which occurs when scientists only report results when the estimated effect sizes are large or are statistically significant. As a result, if many researchers are carrying out studies under low statistical power, the reported results are biased to be stronger than true effects, if any. Another example, where effect sizes may be distorted is in a multiple trial experiment where the effect size calculation is based on the averaged or aggregated response across the trials.
s used in hypothesis testing, in that they estimate the strength of an apparent relationship, rather than assigning a significance
level reflecting whether the relationship could be due to chance. The effect size does not determine the significance level, or vice-versa. Given a sufficiently large sample size, a statistical comparison will always show a significant difference unless the population effect size is exactly zero. For example, a sample Pearson correlation coefficient of 0.1 is strongly statistically significant if the sample size is 1000. Reporting only the significant p-value from this analysis could be misleading if a correlation of 0.1 is too small to be of interest in a particular application.
, often denoted r and introduced by Karl Pearson
, is widely used as an effect size when paired quantitative data are available; for instance if one were studying the relationship between birth weight and longevity. The correlation coefficient can also be used when the data are binary. Pearson's r can vary in magnitude from −1 to 1, with −1 indicating a perfect negative linear relation, 1 indicating a perfect positive linear relation, and 0 indicating no linear relation between two variables. Cohen gives the following guidelines for the social sciences: small effect size, r = 0.1 − 0.23; medium, r = 0.24 − 0.36; large, r = 0.37 or larger.
A related effect size is the coefficient of determination
(the square of r, referred to as "r-squared"). In the case of paired data, this is a measure of the proportion of variance shared by the two variables, and varies from 0 to 1. An r of 0.21, this makes the coefficient of determination .0441, means that 4.4% of the variance of either variable is shared with the other variable. The r2 is positive, so does not convey the polarity of the relationship between the two variables.
where μ1 is the mean for one population, μ2 is the mean for the other population, and σ is a standard deviation
based on either or both populations.
In the practical setting the population values are typically not known and must be estimated from sample statistics. The several versions of effect sizes based on means differ with respect to which statistics are used.
This form for the effect size resembles the computation for a t-test statistic, with the critical difference that the t-test statistic includes a factor of
. This means that for a given effect size, the significance level increases with the sample size. Unlike the t-test statistic, the effect size aims to estimate a population parameter
, so is not affected by the sample size.
Cohen's d is frequently used in estimating sample sizes. A lower Cohen's d indicates a necessity of larger sample sizes, and vice versa, as can subsequently be determined together with the additional parameters of desired significance level and statistical power
.
What precisely the standard deviation s is was not originally made explicit by Jacob Cohen because he defined it (using the symbol "σ") as "the standard deviation of either population (since they are assumed equal)".
Other authors make the computation of the standard deviation more explicit with the following definition for a pooled standard deviation with two independent samples.
This definition of "Cohen's d" is termed the maximum likelihood
estimator by Hedges and Olkin,
and it is related to Hedges' g (see below) by a scaling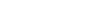
proposed an estimator of the effect size that uses only the standard deviation of the second group
The second group may be regarded as a control group, and Glass argued that if several treatments were compared to the control group it would be better to use just the standard deviation computed from the control group, so that effect sizes would not differ under equal means and different variances.
Under an assumption of equal population variances a pooled estimate for σ is more precise.
is like the other measures based on a standardized difference
but its pooled standard deviation
is computed slightly differently from Cohen's d
As an estimator
for the population effect size θ it is bias
ed.
However, this bias can be corrected for by multiplication with a factor
Hedges and Olkin refer to this unbiased estimator
as d, but it is not the same as Cohen's d.
The exact form for the correction factor J involves the gamma function
distributed a scaled Hedges' g,
, follows a noncentral t-distribution
with the noncentrality parameter
and n1 + n2 − 2 degrees of freedom. Likewise, the scaled Glass' Δ is distributed with n2 − 1 degrees of freedom.
From the distribution it is possible to compute the expectation
and variance of the effect sizes.
In some cases large sample approximations for the variance are used.
One suggestion for the variance of Hedges' unbiased estimator is
for ANOVA or multiple regression. Unfortunately, it estimates for the sample rather than the population and is biased (overestimates effect size for the ANOVA).
The ƒ2 effect size measure for multiple regression is defined as:

The
effect size measure for hierarchical multiple regression is defined as:

By convention, ƒ2A effect sizes of 0.02, 0.15, and 0.35 are termed small, medium, and large, respectively.
Cohen's
can also be found for factorial analysis of variance (ANOVA, aka the F-test) working backwards using :
In a balanced design (equivalent sample sizes across groups) of ANOVA, the corresponding population parameter of
is
wherein μj denotes the population mean within the jth group of the total K groups, and σ the equivalent population standard deviations within each groups. SS is the sum of squares
manipulation in ANOVA. An unbiased estimator for ANOVA would be based on Omega squared, which estimates for the population.

This form of the formula is limited to between-subjects analysis with equal sample sizes in all cells,. Since it is unbiased, ω2 is preferable to Cohen's ƒ2; however, it can be more inconvenient to calculate for complex analyses. A generalized form of the estimator has been published for between-subjects and within-subjects analysis, repeated measure, mixed design, and randomized block design experiments. In addition, methods to calculate partial Omega2 for individual factors and combined factors in designs with up to three independent variables have been published.
The best measure of association for the chi-squared test is phi (or Cramér
's phi or V). Phi is related to the point-biserial correlation coefficient
and Cohen's d and estimates the extent of the relationship between two variables (2 x 2). Cramér's Phi may be used with variables having more than two levels.
Phi can be computed by finding the square root of the chi-squared statistic divided by the sample size.
Similarly, Cramér's phi is computed by taking the square root of the chi-squared statistic divided by the sample size and the length of the minimum dimension (k is the smaller of the number of rows r or columns c).
φc is the intercorrelation of the two discrete variables and may be computed for any value of r or c. However, as chi-squared values tend to increase with the number of cells, the greater the difference between r and c, the more likely φc will tend to 1 without strong evidence of a meaningful correlation.
Cramér's phi may also be applied to 'goodness of fit' chi-squared models (i.e. those where c=1). In this case it functions as a measure of tendency towards a single outcome (i.e. out of k outcomes).
(OR) is another useful effect size. It is appropriate when both variables are binary. For example, consider a study on spelling. In a control group, two students pass the class for every one who fails, so the odds of passing are two to one (or more briefly 2/1 = 2). In the treatment group, six students pass for every one who fails, so the odds of passing are six to one (or 6/1 = 6). The effect size can be computed by noting that the odds of passing in the treatment group are three times higher than in the control group (because 6 divided by 2 is 3). Therefore, the odds ratio is 3. However, odds ratio statistics are on a different scale to Cohen's d. So, this '3' is not comparable to a Cohen's d of 3.
(RR), also called risk ratio, is simply the risk (probability) of an event relative to some independent variable. This measure of effect size differs from the odds ratio in that it compares probabilities instead of odds, but asymptotically approaches the latter for small probabilities. Using the example above, the probabilities for those in the control group and treatment group passing is 2/3 (or 0.67) and 6/7 (or 0.86), respectively. The effect size can be computed the same as above, but using the probabilities instead. Therefore, the relative risk is 1.28. Since rather large probabilities of passing were used, there is a large difference between relative risk and odds ratio. Had failure (a smaller probability) been used as the event (rather than passing), the difference between the two measures of effect size would not be so great.
While both measures are useful, they have different statistical uses. In medical research, the odds ratio
is commonly used for case-control studies, as odds, but not probabilities, are usually estimated. Relative risk is commonly used in randomized controlled trial
s and cohort studies
. When the incidence of outcomes are rare in the study population (generally interpreted to mean less than 10%), the odds ratio is considered a good estimate of the risk ratio. However, as outcomes become more common, the odds ratio and risk ratio diverge, with the odds ratio overestimating or underestimating the risk ratio when the estimates are greater than or less than 1, respectively. When estimates of the incidence of outcomes are available, methods exist to convert odds ratios to risk ratios.
can be found in common statistics textbooks and software, while confidence intervals of standardized effect sizes, especially Cohen's
and
, rely on the calculation of confidence intervals of noncentrality parameters
(ncp). A common approach to construct (1 − α) the confidence interval of ncp is to find the critical ncp values to fit the observed statistic to tail quantiles α/2 and (1 − α/2). SAS and R-package MBESS provide functions for critical values of ncp. See also an online calculator with an interactive interface.


and Cohen's
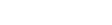
is the point estimate of
So,
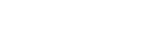
wherein

and Cohen's
So,
, the same test question applies noncentral chi-squared distribution.
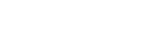
For each j-th sample within i-th group Xi,j, denote
While,

So, both ncp(s) of F and
equate

In case of
for K independent groups of same size, the total sample size is N := n·K.

T-test of pair of independent groups is a special case of one-way ANOVA. Note that noncentrality parameter
of F is not comparable to the noncentrality parameter
of the corresponding t. Actually,
, and
in the case.
Whether an effect size should be interpreted small, medium, or large depends on its substantial context and its operational definition. Cohen's conventional criteria small, medium, or big are near ubiquitous across many fields. Power analysis or sample size planning requires an assumed population parameter of effect sizes. Many researchers adopt Cohen's standards as default alternative hypotheses. Russell Lenth criticized them as T-shirt effect sizes
For Cohen's d an effect size of 0.2 to 0.3 might be a "small" effect, around 0.5 a "medium" effect and 0.8 to infinity, a "large" effect.
(But note that the d might be larger than one)
Cohen's text anticipates Lenth's concerns:
In an ideal world, researchers would interpret the substantive significance of their results by grounding them in a meaningful context or by quantifying their contribution to knowledge. Where this is problematic, Cohen's effect size criteria may serve as a last resort.
Further Explanations
Statistics
Statistics is the study of the collection, organization, analysis, and interpretation of data. It deals with all aspects of this, including the planning of data collection in terms of the design of surveys and experiments....
, an effect size is a measure of the strength of the relationship between two variables in a statistical population
Statistical population
A statistical population is a set of entities concerning which statistical inferences are to be drawn, often based on a random sample taken from the population. For example, if we were interested in generalizations about crows, then we would describe the set of crows that is of interest...
, or a sample-based estimate of that quantity. An effect size calculated from data
Data
The term data refers to qualitative or quantitative attributes of a variable or set of variables. Data are typically the results of measurements and can be the basis of graphs, images, or observations of a set of variables. Data are often viewed as the lowest level of abstraction from which...
is a descriptive statistic
Descriptive statistics
Descriptive statistics quantitatively describe the main features of a collection of data. Descriptive statistics are distinguished from inferential statistics , in that descriptive statistics aim to summarize a data set, rather than use the data to learn about the population that the data are...
that conveys the estimated magnitude of a relationship without making any statement about whether the apparent relationship in the data reflects a true relationship in the population
Statistical population
A statistical population is a set of entities concerning which statistical inferences are to be drawn, often based on a random sample taken from the population. For example, if we were interested in generalizations about crows, then we would describe the set of crows that is of interest...
. In that way, effect sizes complement inferential statistics such as p-values. Among other uses, effect size measures play an important role in meta-analysis
Meta-analysis
In statistics, a meta-analysis combines the results of several studies that address a set of related research hypotheses. In its simplest form, this is normally by identification of a common measure of effect size, for which a weighted average might be the output of a meta-analyses. Here the...
studies that summarize findings from a specific area of research, and in statistical power
Statistical power
The power of a statistical test is the probability that the test will reject the null hypothesis when the null hypothesis is actually false . The power is in general a function of the possible distributions, often determined by a parameter, under the alternative hypothesis...
analyses.
The concept of effect size appears already in everyday language. For example, a weight loss program may boast that it leads to an average weight loss of 30 pounds. In this case, 30 pounds is an indicator of the claimed effect size. Another example is that a tutoring program may claim that it raises school performance by one letter grade. This grade increase is the claimed effect size of the program. These are both examples of "absolute effect sizes," meaning that they convey the average difference between two groups without any discussion of the variability
Variance
In probability theory and statistics, the variance is a measure of how far a set of numbers is spread out. It is one of several descriptors of a probability distribution, describing how far the numbers lie from the mean . In particular, the variance is one of the moments of a distribution...
within the groups. For example, if the weight loss program results in an average loss of 30 pounds, it is possible that every participant loses exactly 30 pounds, or half the participants lose 60 pounds and half lose no weight at all.
Reporting effect sizes is considered good practice when presenting empirical research findings in many fields. The reporting of effect sizes facilitates the interpretation of the substantive, as opposed to the statistical, significance of a research result.
Effect sizes are particularly prominent in social and medical research. Relative and absolute measures of effect size convey different information, and can be used complementarily. A prominent task force in the psychology research community expressed the following recommendation:
Population and sample effect sizes
The term effect size can refer to a statistic calculated from a sample of dataData
The term data refers to qualitative or quantitative attributes of a variable or set of variables. Data are typically the results of measurements and can be the basis of graphs, images, or observations of a set of variables. Data are often viewed as the lowest level of abstraction from which...
, or to a parameter of a hypothetical statistical population. Conventions for distinguishing sample from population effect sizes follow standard statistical practices — one common approach is to use Greek letters like ρ to denote population parameters and Latin letters like r to denote the corresponding statistic; alternatively, a "hat" can be placed over the population parameter to denote the statistic, e.g. with ρ̂ being the estimate of the parameter ρ.
As in any statistical setting, effect sizes are estimated with error, and may be biased unless the effect size estimator that is used is appropriate for the manner in which the data were sampled
Sampling (statistics)
In statistics and survey methodology, sampling is concerned with the selection of a subset of individuals from within a population to estimate characteristics of the whole population....
and the manner in which the measurements were made. An example of this is publication bias
Publication bias
Publication bias is the tendency of researchers, editors, and pharmaceutical companies to handle the reporting of experimental results that are positive differently from results that are negative or inconclusive, leading to bias in the overall published literature...
, which occurs when scientists only report results when the estimated effect sizes are large or are statistically significant. As a result, if many researchers are carrying out studies under low statistical power, the reported results are biased to be stronger than true effects, if any. Another example, where effect sizes may be distorted is in a multiple trial experiment where the effect size calculation is based on the averaged or aggregated response across the trials.
Relationship to test statistics
Sample-based effect sizes are distinguished from test statisticTest statistic
In statistical hypothesis testing, a hypothesis test is typically specified in terms of a test statistic, which is a function of the sample; it is considered as a numerical summary of a set of data that...
s used in hypothesis testing, in that they estimate the strength of an apparent relationship, rather than assigning a significance
Statistical significance
In statistics, a result is called statistically significant if it is unlikely to have occurred by chance. The phrase test of significance was coined by Ronald Fisher....
level reflecting whether the relationship could be due to chance. The effect size does not determine the significance level, or vice-versa. Given a sufficiently large sample size, a statistical comparison will always show a significant difference unless the population effect size is exactly zero. For example, a sample Pearson correlation coefficient of 0.1 is strongly statistically significant if the sample size is 1000. Reporting only the significant p-value from this analysis could be misleading if a correlation of 0.1 is too small to be of interest in a particular application.
Standardized and unstandardized effect sizes
The term effect size can refer to a standardized measures of effect (such as r, Cohen's d, and odds ratio), or to an unstandardized measure (e.g., the raw difference between group means and unstandardized regression coefficients). Standardized effect size measures are typically used when the metrics of variables being studied do not have intrinsic meaning (e.g., a score on a personality test on an arbitrary scale), when results from multiple studies are being combined when some or all of the studies use different scales, or when it is desired to convey the size of an effect relative to the variability in the population. In meta-analysis, standardized effect sizes are used as a common measure that can be calculated for different studies and then combined into an overall summary.Pearson r correlation
Pearson's correlationPearson product-moment correlation coefficient
In statistics, the Pearson product-moment correlation coefficient is a measure of the correlation between two variables X and Y, giving a value between +1 and −1 inclusive...
, often denoted r and introduced by Karl Pearson
Karl Pearson
Karl Pearson FRS was an influential English mathematician who has been credited for establishing the disciplineof mathematical statistics....
, is widely used as an effect size when paired quantitative data are available; for instance if one were studying the relationship between birth weight and longevity. The correlation coefficient can also be used when the data are binary. Pearson's r can vary in magnitude from −1 to 1, with −1 indicating a perfect negative linear relation, 1 indicating a perfect positive linear relation, and 0 indicating no linear relation between two variables. Cohen gives the following guidelines for the social sciences: small effect size, r = 0.1 − 0.23; medium, r = 0.24 − 0.36; large, r = 0.37 or larger.
A related effect size is the coefficient of determination
Coefficient of determination
In statistics, the coefficient of determination R2 is used in the context of statistical models whose main purpose is the prediction of future outcomes on the basis of other related information. It is the proportion of variability in a data set that is accounted for by the statistical model...
(the square of r, referred to as "r-squared"). In the case of paired data, this is a measure of the proportion of variance shared by the two variables, and varies from 0 to 1. An r of 0.21, this makes the coefficient of determination .0441, means that 4.4% of the variance of either variable is shared with the other variable. The r2 is positive, so does not convey the polarity of the relationship between the two variables.
Effect sizes based on means
A (population) effect size θ based on means usually considers the standardized mean difference between two populationswhere μ1 is the mean for one population, μ2 is the mean for the other population, and σ is a standard deviation
Standard deviation
Standard deviation is a widely used measure of variability or diversity used in statistics and probability theory. It shows how much variation or "dispersion" there is from the average...
based on either or both populations.
In the practical setting the population values are typically not known and must be estimated from sample statistics. The several versions of effect sizes based on means differ with respect to which statistics are used.
This form for the effect size resembles the computation for a t-test statistic, with the critical difference that the t-test statistic includes a factor of

Parameter
Parameter from Ancient Greek παρά also “para” meaning “beside, subsidiary” and μέτρον also “metron” meaning “measure”, can be interpreted in mathematics, logic, linguistics, environmental science and other disciplines....
, so is not affected by the sample size.
Cohen's d
Cohen's d is defined as the difference between two means divided by a standard deviation for the dataCohen's d is frequently used in estimating sample sizes. A lower Cohen's d indicates a necessity of larger sample sizes, and vice versa, as can subsequently be determined together with the additional parameters of desired significance level and statistical power
Statistical power
The power of a statistical test is the probability that the test will reject the null hypothesis when the null hypothesis is actually false . The power is in general a function of the possible distributions, often determined by a parameter, under the alternative hypothesis...
.
What precisely the standard deviation s is was not originally made explicit by Jacob Cohen because he defined it (using the symbol "σ") as "the standard deviation of either population (since they are assumed equal)".
Other authors make the computation of the standard deviation more explicit with the following definition for a pooled standard deviation with two independent samples.
This definition of "Cohen's d" is termed the maximum likelihood
Maximum likelihood
In statistics, maximum-likelihood estimation is a method of estimating the parameters of a statistical model. When applied to a data set and given a statistical model, maximum-likelihood estimation provides estimates for the model's parameters....
estimator by Hedges and Olkin,
and it is related to Hedges' g (see below) by a scaling
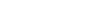
Glass's Δ
In 1976 Gene V. GlassGene V. Glass
Gene V Glass is an American statistician and researcher working in educational psychology and the social sciences. He coined the term "meta-analysis" and illustrated its use in 1976 while a faculty member at the University of Colorado Boulder...
proposed an estimator of the effect size that uses only the standard deviation of the second group
The second group may be regarded as a control group, and Glass argued that if several treatments were compared to the control group it would be better to use just the standard deviation computed from the control group, so that effect sizes would not differ under equal means and different variances.
Under an assumption of equal population variances a pooled estimate for σ is more precise.
Hedges' g
Hedges' g, suggested by Larry Hedges in 1981,is like the other measures based on a standardized difference
but its pooled standard deviation

As an estimator
Estimator
In statistics, an estimator is a rule for calculating an estimate of a given quantity based on observed data: thus the rule and its result are distinguished....
for the population effect size θ it is bias
Bias of an estimator
In statistics, bias of an estimator is the difference between this estimator's expected value and the true value of the parameter being estimated. An estimator or decision rule with zero bias is called unbiased. Otherwise the estimator is said to be biased.In ordinary English, the term bias is...
ed.
However, this bias can be corrected for by multiplication with a factor
Hedges and Olkin refer to this unbiased estimator

The exact form for the correction factor J involves the gamma function
Gamma function
In mathematics, the gamma function is an extension of the factorial function, with its argument shifted down by 1, to real and complex numbers...
Distribution of effect sizes based on means
Provided that the data is GaussianGAUSSIAN
Gaussian is a computational chemistry software program initially released in 1970 by John Pople and his research group at Carnegie-Mellon University as Gaussian 70. It has been continuously updated since then...
distributed a scaled Hedges' g,

Noncentral t-distribution
In probability and statistics, the noncentral t-distribution generalizes Student's t-distribution using a noncentrality parameter. Like the central t-distribution, the noncentral t-distribution is primarily used in statistical inference, although it may also be used in robust modeling for data...
with the noncentrality parameter
Noncentrality parameter
Noncentrality parameters are parameters of families of probability distributions which are related to other "central" families of distributions. If the noncentrality parameter of a distribution is zero, the distribution is identical to a distribution in the central family...

From the distribution it is possible to compute the expectation
Expectation
In the case of uncertainty, expectation is what is considered the most likely to happen. An expectation, which is a belief that is centered on the future, may or may not be realistic. A less advantageous result gives rise to the emotion of disappointment. If something happens that is not at all...
and variance of the effect sizes.
In some cases large sample approximations for the variance are used.
One suggestion for the variance of Hedges' unbiased estimator is
Cohen's ƒ2
Cohen's ƒ2 is one of several effect size measures to use in the context of an F-testF-test
An F-test is any statistical test in which the test statistic has an F-distribution under the null hypothesis.It is most often used when comparing statistical models that have been fit to a data set, in order to identify the model that best fits the population from which the data were sampled. ...
for ANOVA or multiple regression. Unfortunately, it estimates for the sample rather than the population and is biased (overestimates effect size for the ANOVA).
The ƒ2 effect size measure for multiple regression is defined as:

- where R2 is the squared multiple correlation.
The


- where R2A is the variance accounted for by a set of one or more independent variables A, and R2AB is the combined variance accounted for by A and another set of one or more independent variables B.
By convention, ƒ2A effect sizes of 0.02, 0.15, and 0.35 are termed small, medium, and large, respectively.
Cohen's

In a balanced design (equivalent sample sizes across groups) of ANOVA, the corresponding population parameter of

wherein μj denotes the population mean within the jth group of the total K groups, and σ the equivalent population standard deviations within each groups. SS is the sum of squares
Sum of squares
The partition of sums of squares is a concept that permeates much of inferential statistics and descriptive statistics. More properly, it is the partitioning of sums of squared deviations or errors. Mathematically, the sum of squared deviations is an unscaled, or unadjusted measure of dispersion...
manipulation in ANOVA. An unbiased estimator for ANOVA would be based on Omega squared, which estimates for the population.
ω2
A more unbiased estimator of the variance explained in the population is omega-squared
This form of the formula is limited to between-subjects analysis with equal sample sizes in all cells,. Since it is unbiased, ω2 is preferable to Cohen's ƒ2; however, it can be more inconvenient to calculate for complex analyses. A generalized form of the estimator has been published for between-subjects and within-subjects analysis, repeated measure, mixed design, and randomized block design experiments. In addition, methods to calculate partial Omega2 for individual factors and combined factors in designs with up to three independent variables have been published.
φ, Cramér's φ, or Cramér's V
![]() |
![]() |
Phi (φ) | Cramér's Phi (φc) |
---|
The best measure of association for the chi-squared test is phi (or Cramér
Harald Cramér
Harald Cramér was a Swedish mathematician, actuary, and statistician, specializing in mathematical statistics and probabilistic number theory. He was once described by John Kingman as "one of the giants of statistical theory".-Early life:Harald Cramér was born in Stockholm, Sweden on September...
's phi or V). Phi is related to the point-biserial correlation coefficient
Point-biserial correlation coefficient
The point biserial correlation coefficient is a correlation coefficient used when one variable is dichotomous; Y can either be "naturally" dichotomous, like gender, or an artificially dichotomized variable. In most situations it is not advisable to artificially dichotomize variables...
and Cohen's d and estimates the extent of the relationship between two variables (2 x 2). Cramér's Phi may be used with variables having more than two levels.
Phi can be computed by finding the square root of the chi-squared statistic divided by the sample size.
Similarly, Cramér's phi is computed by taking the square root of the chi-squared statistic divided by the sample size and the length of the minimum dimension (k is the smaller of the number of rows r or columns c).
φc is the intercorrelation of the two discrete variables and may be computed for any value of r or c. However, as chi-squared values tend to increase with the number of cells, the greater the difference between r and c, the more likely φc will tend to 1 without strong evidence of a meaningful correlation.
Cramér's phi may also be applied to 'goodness of fit' chi-squared models (i.e. those where c=1). In this case it functions as a measure of tendency towards a single outcome (i.e. out of k outcomes).
Odds ratio
The odds ratioOdds ratio
The odds ratio is a measure of effect size, describing the strength of association or non-independence between two binary data values. It is used as a descriptive statistic, and plays an important role in logistic regression...
(OR) is another useful effect size. It is appropriate when both variables are binary. For example, consider a study on spelling. In a control group, two students pass the class for every one who fails, so the odds of passing are two to one (or more briefly 2/1 = 2). In the treatment group, six students pass for every one who fails, so the odds of passing are six to one (or 6/1 = 6). The effect size can be computed by noting that the odds of passing in the treatment group are three times higher than in the control group (because 6 divided by 2 is 3). Therefore, the odds ratio is 3. However, odds ratio statistics are on a different scale to Cohen's d. So, this '3' is not comparable to a Cohen's d of 3.
Relative risk
The relative riskRelative risk
In statistics and mathematical epidemiology, relative risk is the risk of an event relative to exposure. Relative risk is a ratio of the probability of the event occurring in the exposed group versus a non-exposed group....
(RR), also called risk ratio, is simply the risk (probability) of an event relative to some independent variable. This measure of effect size differs from the odds ratio in that it compares probabilities instead of odds, but asymptotically approaches the latter for small probabilities. Using the example above, the probabilities for those in the control group and treatment group passing is 2/3 (or 0.67) and 6/7 (or 0.86), respectively. The effect size can be computed the same as above, but using the probabilities instead. Therefore, the relative risk is 1.28. Since rather large probabilities of passing were used, there is a large difference between relative risk and odds ratio. Had failure (a smaller probability) been used as the event (rather than passing), the difference between the two measures of effect size would not be so great.
While both measures are useful, they have different statistical uses. In medical research, the odds ratio
Odds ratio
The odds ratio is a measure of effect size, describing the strength of association or non-independence between two binary data values. It is used as a descriptive statistic, and plays an important role in logistic regression...
is commonly used for case-control studies, as odds, but not probabilities, are usually estimated. Relative risk is commonly used in randomized controlled trial
Randomized controlled trial
A randomized controlled trial is a type of scientific experiment - a form of clinical trial - most commonly used in testing the safety and efficacy or effectiveness of healthcare services or health technologies A randomized controlled trial (RCT) is a type of scientific experiment - a form of...
s and cohort studies
Cohort study
A cohort study or panel study is a form of longitudinal study used in medicine, social science, actuarial science, and ecology. It is an analysis of risk factors and follows a group of people who do not have the disease, and uses correlations to determine the absolute risk of subject contraction...
. When the incidence of outcomes are rare in the study population (generally interpreted to mean less than 10%), the odds ratio is considered a good estimate of the risk ratio. However, as outcomes become more common, the odds ratio and risk ratio diverge, with the odds ratio overestimating or underestimating the risk ratio when the estimates are greater than or less than 1, respectively. When estimates of the incidence of outcomes are available, methods exist to convert odds ratios to risk ratios.
Confidence intervals by means of noncentrality parameters
Confidence intervals of unstandardized effect sizes like difference of means


Noncentrality parameter
Noncentrality parameters are parameters of families of probability distributions which are related to other "central" families of distributions. If the noncentrality parameter of a distribution is zero, the distribution is identical to a distribution in the central family...
(ncp). A common approach to construct (1 − α) the confidence interval of ncp is to find the critical ncp values to fit the observed statistic to tail quantiles α/2 and (1 − α/2). SAS and R-package MBESS provide functions for critical values of ncp. See also an online calculator with an interactive interface.
T test for mean difference of single group or two related groups
In case of single group, M (μ) denotes the sample (population) mean of single group, and SD (σ) denotes the sample (population) standard deviation. N is the sample size of the group. T test is used for the hypothesis on the difference between mean and a baseline μbaseline. Usually, μbaseline is zero, while not necessary. In case of two related groups, the single group is constructed by difference in each pair of samples, while SD (σ) denotes the sample (population) standard deviation of differences rather than within original two groups.

and Cohen's
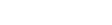
is the point estimate of
So,

T test for mean difference between two independent groups
n1 or n2 is sample size within the respective group.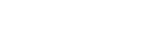
wherein

and Cohen's
-
is the point estimate of
So,

One-way ANOVA test for mean difference across multiple independent groups
One-way ANOVA test applies noncentral F distribution. While with a given population standard deviation
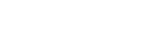
For each j-th sample within i-th group Xi,j, denote
While,

So, both ncp(s) of F and


In case of


T-test of pair of independent groups is a special case of one-way ANOVA. Note that noncentrality parameter




"Small", "medium", "large"
Some fields using effect sizes apply words such as "small", "medium" and "large" to the size of the effect.Whether an effect size should be interpreted small, medium, or large depends on its substantial context and its operational definition. Cohen's conventional criteria small, medium, or big are near ubiquitous across many fields. Power analysis or sample size planning requires an assumed population parameter of effect sizes. Many researchers adopt Cohen's standards as default alternative hypotheses. Russell Lenth criticized them as T-shirt effect sizes
This is an elaborate way to arrive at the same sample size that has been used in past social science studies of large, medium, and small size (respectively). The method uses a standardized effect size as the goal. Think about it: for a "medium" effect size, you'll choose the same n regardless of the accuracy or reliability of your instrument, or the narrowness or diversity of your subjects. Clearly, important considerations are being ignored here. "Medium" is definitely not the message!
For Cohen's d an effect size of 0.2 to 0.3 might be a "small" effect, around 0.5 a "medium" effect and 0.8 to infinity, a "large" effect.
(But note that the d might be larger than one)
Cohen's text anticipates Lenth's concerns:
"The terms 'small,' 'medium,' and 'large' are relative, not only to each other, but to the area of behavioral science or even more particularly to the specific content and research method being employed in any given investigation....In the face of this relativity, there is a certain risk inherent in offering conventional operational definitions for these terms for use in power analysis in as diverse a field of inquiry as behavioral science. This risk is nevertheless accepted in the belief that more is to be gained than lost by supplying a common conventional frame of reference which is recommended for use only when no better basis for estimating the ES index is available." (p. 25)
In an ideal world, researchers would interpret the substantive significance of their results by grounding them in a meaningful context or by quantifying their contribution to knowledge. Where this is problematic, Cohen's effect size criteria may serve as a last resort.
Further reading
- Aaron, B., Kromrey, J. D., & Ferron, J. M. (1998, November). Equating r-based and d-based effect-size indices: Problems with a commonly recommended formula. Paper presented at the annual meeting of the Florida Educational Research Association, Orlando, FL. (ERIC Document Reproduction Service No. ED433353)
- Bonett, D.G. (2008). Confidence intervals for standardized linear contrasts of means, Psychological Methods, 13, 99-109.
- Cumming, G. and Finch, S. (2001). A primer on the understanding, use, and calculation of confidence intervals that are based on central and noncentral distributions. Educational and Psychological Measurement, 61, 530–572.
- Kelley, K. (2007). Confidence intervals for standardized effect sizes: Theory, application, and implementation. Journal of Statistical Software, 20(8), 1-24. http://www.jstatsoft.org/v20/i08/paper
- Lipsey, M.W., & Wilson, D.B. (2001). Practical meta-analysis. Sage: Thousand Oaks, CA.
External links
Software- MIX 2.0 Software for professional meta-analysis in Excel. Many effect sizes available.
- Effect Size Calculators Calculate d and r from a variety of statistics.
- Free Effect Size Generator - PC & Mac Software
- MBESS - One of R's packagesR (programming language)R is a programming language and software environment for statistical computing and graphics. The R language is widely used among statisticians for developing statistical software, and R is widely used for statistical software development and data analysis....
providing confidence intervals of effect sizes based non-central parameters - Free GPower Software - PC & Mac Software
- Free Effect Size Calculator for Multiple Regression - Web Based
- Free Effect Size Calculator for Hierarchical Multiple Regression - Web Based
- Copylefted Online Calculator for Noncentral t, Chisquare, and F Distributions - Collaborated Wiki Page Powered by R
- ES-Calc: a free add-on for Effect Size Calculation in ViSta 'The Visual Statistics System'. Computes Cohen's d, Glass's Delta, Hedges' g, CLES, Non-Parametric Cliff's Delta, d-to-r Conversion, etc.
Further Explanations