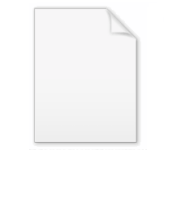
Inductive bias
Encyclopedia
The inductive bias of a learning algorithm
is the set of assumptions that the learner uses to predict outputs given inputs that it has not encountered (Mitchell, 1980).
In machine learning
, one aims to construct algorithms that are able to learn to predict a certain target output. To achieve this, the learning algorithm is presented some training examples that demonstrate the intended relation of input and output values. Then the learner is supposed to approximate the correct output, even for examples that have not been shown during training. Without any additional assumptions, this task cannot be solved exactly since unseen situations might have an arbitrary output value. The kind of necessary assumptions about the nature of the target function are subsumed in the term inductive bias (Mitchell, 1980; desJardins and Gordon, 1995).
A classical example of an inductive bias is Occam's Razor
, assuming that the simplest consistent hypothesis about the target function is actually the best. Here consistent means that the hypothesis of the learner yields correct outputs for all of the examples that have been given to the algorithm.
Approaches to a more formal definition of inductive bias are based on mathematical logic
. Here, the inductive bias is a logical formula that, together with the training data, logically entails the hypothesis generated by the learner. Unfortunately, this strict formalism fails in many practical cases, where the inductive bias can only be given as a rough description (e.g. in the case of neural networks
), or not at all.
Algorithm
In mathematics and computer science, an algorithm is an effective method expressed as a finite list of well-defined instructions for calculating a function. Algorithms are used for calculation, data processing, and automated reasoning...
is the set of assumptions that the learner uses to predict outputs given inputs that it has not encountered (Mitchell, 1980).
In machine learning
Machine learning
Machine learning, a branch of artificial intelligence, is a scientific discipline concerned with the design and development of algorithms that allow computers to evolve behaviors based on empirical data, such as from sensor data or databases...
, one aims to construct algorithms that are able to learn to predict a certain target output. To achieve this, the learning algorithm is presented some training examples that demonstrate the intended relation of input and output values. Then the learner is supposed to approximate the correct output, even for examples that have not been shown during training. Without any additional assumptions, this task cannot be solved exactly since unseen situations might have an arbitrary output value. The kind of necessary assumptions about the nature of the target function are subsumed in the term inductive bias (Mitchell, 1980; desJardins and Gordon, 1995).
A classical example of an inductive bias is Occam's Razor
Occam's razor
Occam's razor, also known as Ockham's razor, and sometimes expressed in Latin as lex parsimoniae , is a principle that generally recommends from among competing hypotheses selecting the one that makes the fewest new assumptions.-Overview:The principle is often summarized as "simpler explanations...
, assuming that the simplest consistent hypothesis about the target function is actually the best. Here consistent means that the hypothesis of the learner yields correct outputs for all of the examples that have been given to the algorithm.
Approaches to a more formal definition of inductive bias are based on mathematical logic
Logic
In philosophy, Logic is the formal systematic study of the principles of valid inference and correct reasoning. Logic is used in most intellectual activities, but is studied primarily in the disciplines of philosophy, mathematics, semantics, and computer science...
. Here, the inductive bias is a logical formula that, together with the training data, logically entails the hypothesis generated by the learner. Unfortunately, this strict formalism fails in many practical cases, where the inductive bias can only be given as a rough description (e.g. in the case of neural networks
Neural Networks
Neural Networks is the official journal of the three oldest societies dedicated to research in neural networks: International Neural Network Society, European Neural Network Society and Japanese Neural Network Society, published by Elsevier...
), or not at all.
Types of inductive biases
The following is a list of common inductive biases in machine learning algorithms.- Maximum conditional independenceConditional independenceIn probability theory, two events R and B are conditionally independent given a third event Y precisely if the occurrence or non-occurrence of R and the occurrence or non-occurrence of B are independent events in their conditional probability distribution given Y...
: if the hypothesis can be cast in a BayesianBayesian inferenceIn statistics, Bayesian inference is a method of statistical inference. It is often used in science and engineering to determine model parameters, make predictions about unknown variables, and to perform model selection...
framework, try to maximize conditional independence. This is the bias used in the Naive Bayes classifierNaive Bayes classifierA naive Bayes classifier is a simple probabilistic classifier based on applying Bayes' theorem with strong independence assumptions...
. - Minimum cross-validation error: when trying to choose among hypotheses, select the hypothesis with the lowest cross-validation error. Although cross-validation may seem to be free of bias, the No Free Lunch theorems show that cross-validation must be biased.
- Maximum margin: when drawing a boundary between two classes, attempt to maximize the width of the boundary. This is the bias used in Support Vector Machines. The assumption is that distinct classes tend to be separated by wide boundaries.
- Minimum description lengthMinimum description lengthThe minimum description length principle is a formalization of Occam's Razor in which the best hypothesis for a given set of data is the one that leads to the best compression of the data. MDL was introduced by Jorma Rissanen in 1978...
: when forming a hypothesis, attempt to minimize the length of the description of the hypothesis. The assumption is that simpler hypotheses are more likely to be true. See Occam's razorOccam's razorOccam's razor, also known as Ockham's razor, and sometimes expressed in Latin as lex parsimoniae , is a principle that generally recommends from among competing hypotheses selecting the one that makes the fewest new assumptions.-Overview:The principle is often summarized as "simpler explanations...
. - Minimum features: unless there is good evidence that a featureFeature spaceIn pattern recognition a feature space is an abstract space where each pattern sample is represented as a point in n-dimensional space. Its dimension is determined by the number of features used to describe the patterns...
is useful, it should be deleted. This is the assumption behind feature selectionFeature selectionIn machine learning and statistics, feature selection, also known as variable selection, feature reduction, attribute selection or variable subset selection, is the technique of selecting a subset of relevant features for building robust learning models...
algorithms. - Nearest neighbors: assume that most of the cases in a small neighborhood in feature spaceFeature spaceIn pattern recognition a feature space is an abstract space where each pattern sample is represented as a point in n-dimensional space. Its dimension is determined by the number of features used to describe the patterns...
belong to the same class. Given a case for which the class is unknown, guess that it belongs to the same class as the majority in its immediate neighborhood. This is the bias used in the k-nearest neighbor algorithmK-nearest neighbor algorithmIn pattern recognition, the k-nearest neighbor algorithm is a method for classifying objects based on closest training examples in the feature space. k-NN is a type of instance-based learning, or lazy learning where the function is only approximated locally and all computation is deferred until...
. The assumption is that cases that are near each other tend to belong to the same class.