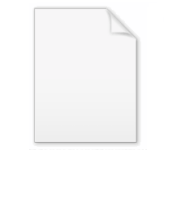
Activity recognition
Encyclopedia
Activity recognition aims to recognize the actions and goals of one or more agents from a series of observations on the agents' actions and the environmental conditions. Since the 1980s, this research field has captured the attention of several computer science
communities due to its strength in providing personalized support for many different applications and its connection to many different fields of study such as medicine, human-computer interaction, or sociology.
To understand activity recognition better, consider the following scenario. An elderly man wakes up at dawn in his small studio apartment, where he stays alone. He lights the stove to make a pot of tea, switches on the toaster oven, and takes some bread and jelly from the cupboard. After taking his morning medication, a computer-generated voice gently reminds him to turn off the toaster. Later that day, his daughter accesses a secure website where she scans a check-list, which was created by a sensor network in her father's apartment. She finds that her father is eating normally, taking his medicine on schedule, and continuing to manage his daily life on his own. That information puts her mind at ease.
Many different applications have been studied by researchers in activity recognition; examples include assisting the sick and disabled. For example, Pollack et al. show that by automatically monitoring human activities, home-based rehabilitation can be provided for people suffering from traumatic brain injuries. One can find applications ranging from security-related applications and logistics support to location-based service
s. Due to its many-faceted nature, different fields may refer to activity recognition as plan recognition, goal recognition, intent recognition, behavior recognition, location estimation and location-based services.
-based activity recognition integrates the emerging area of sensor networks with novel data mining
and machine learning
techniques to model a wide range of human activities. Mobile devices (e.g. smart phones) provide sufficient sensor data and calculation power to enable physical activity recognition to provide an estimation of the energy consumption during everyday life. Sensor-based activity recognition researchers believe that by empowering ubiquitous computers
and sensors to monitor the behavior of agents (under consent), these computers will be better suited to act on our behalf.
may be concerned about how to recognize individuals' activities from the inferred location sequences and environmental conditions at the lower levels. Furthermore, at the highest level a major concern is to find out the overall goal or subgoals of an agent from the activity sequences through a mixture of logical and statistical reasoning. Scientific conferences where activity recognition work from wearable and environmental often appears are ISWC
and UbiComp.
Scientific conferences where vision based activity recognition work often appears are ICCV and CVPR.
In vision-based activity recognition, a great deal of work has been done. Researchers have attempted a number of methods such as optical flow
, Kalman filtering, hidden Markov model
s, etc., under different modalities such as single camera, stereo, and infrared. In addition, researchers have considered multiple aspects on this topic, including single pedestrian tracking, group tracking, and detecting dropped objects.
Kautz's general framework for plan recognition has an exponential time complexity in worst case, measured in the size of input hierarchy. Lesh and Etzioni went one step further and presented methods in scaling up goal recognition to scale up his work computationally. In contrast to Kautz's approach where the plan library is explicitly represented, Lesh and Etzioni's approach enables automatic plan-library construction from domain primitives. Furthermore, they introduced compact representations and efficient algorithms for goal recognition on large plan libraries.
Inconsistent plans and goals are repeatedly pruned when new actions arrive. Besides, they also presented methods for adapting a goal recognizer to handle individual idiosyncratic behavior given a sample of an individual's recent behavior. Pollack et al. described a direct argumentation model that can know about the relative strength of several kinds of arguments for belief and intention description.
A serious problem of logic-based approaches is their inability or inherent infeasibility to represent uncertainty. They offer no mechanism for preferring one consistent approach to another and incapable of deciding whether one particular plan is more likely than another, as long as both of them can be consistent enough to explain the actions observed. There is also a lack of learning ability associated with logic based methods.
Plan recognition can be done as a process of reasoning under uncertainty, which is convincingly argued by Charniak and Goldman. They argued that any model that does not incorporate some theory of uncertainty reasoning cannot be adequate. In the literature, there have been several approaches which explicitly represent uncertainty in reasoning about an agent's plans and goals.
Using sensor data as input, Hodges and Pollack designed machine learning-based systems for identifying individuals as they perform routine daily activities such as making coffee. Intel Research (Seattle) Lab and University of Washington at Seattle have done some important works on using sensors to detect human plans. Some of these works infer user transportation modes from readings of radio-frequency identifiers (RFID) and global positioning systems (GPS).
signals and 802.11 access points, there is much noise and uncertainty. These uncertainties are modeled using a dynamic Bayesian network
model by Yin et al. A multiple goal model that can reason about user's interleaving goals is presented by Chai and Yang, where a deterministic state transition model is applied. A better model that models the concurrent and interleaving activities in a probabilistic approach is proposed by Hu and Yang. A user action discovery model is presented by Yin et al., where the Wi-Fi signals are segmented to produce possible actions.
A fundamental problem in Wi-Fi-based activity recognition is to estimate the user locations. Two important issues are how to reduce the human labelling effort and how to cope with the changing signal profiles when the environment changes. Yin et al. dealt with the second issue by transferring the labelled knowledge between time periods. Chai and Yang proposed a hidden Markov model-based method to extend labelled knowledge by leveraging the unlabelled user traces. J. Pan et al. propose to perform location estimation through online co-localization, and S. Pan et al. proposed to apply multi-view learning for migrating the labelled data to a new time period.
In the work of Gu et al., the problem of activity recognition is formulated as a pattern-based classification problem. They proposed a data mining approach based on discriminative patterns which describe significant changes between any two activity classes of data to recognize sequential, interleaved and concurrent activities in a unified solution.
Gilbert et al use 2D corners in both space and time. These are grouped spatially and temporally using a hierarchical process, with an increasing search area. At each stage of the hierarchy, the most distinctive and descriptive features are learned efficiently through data mining (Apriori rule).
Computer science
Computer science or computing science is the study of the theoretical foundations of information and computation and of practical techniques for their implementation and application in computer systems...
communities due to its strength in providing personalized support for many different applications and its connection to many different fields of study such as medicine, human-computer interaction, or sociology.
To understand activity recognition better, consider the following scenario. An elderly man wakes up at dawn in his small studio apartment, where he stays alone. He lights the stove to make a pot of tea, switches on the toaster oven, and takes some bread and jelly from the cupboard. After taking his morning medication, a computer-generated voice gently reminds him to turn off the toaster. Later that day, his daughter accesses a secure website where she scans a check-list, which was created by a sensor network in her father's apartment. She finds that her father is eating normally, taking his medicine on schedule, and continuing to manage his daily life on his own. That information puts her mind at ease.
Many different applications have been studied by researchers in activity recognition; examples include assisting the sick and disabled. For example, Pollack et al. show that by automatically monitoring human activities, home-based rehabilitation can be provided for people suffering from traumatic brain injuries. One can find applications ranging from security-related applications and logistics support to location-based service
Location-based service
A Location-Based Service is an information or entertainment service, accessible with mobile devices through the mobile network and utilizing the ability to make use of the geographical position of the mobile device....
s. Due to its many-faceted nature, different fields may refer to activity recognition as plan recognition, goal recognition, intent recognition, behavior recognition, location estimation and location-based services.
Sensor-based, single-user activity recognition
SensorSensor
A sensor is a device that measures a physical quantity and converts it into a signal which can be read by an observer or by an instrument. For example, a mercury-in-glass thermometer converts the measured temperature into expansion and contraction of a liquid which can be read on a calibrated...
-based activity recognition integrates the emerging area of sensor networks with novel data mining
Data mining
Data mining , a relatively young and interdisciplinary field of computer science is the process of discovering new patterns from large data sets involving methods at the intersection of artificial intelligence, machine learning, statistics and database systems...
and machine learning
Machine learning
Machine learning, a branch of artificial intelligence, is a scientific discipline concerned with the design and development of algorithms that allow computers to evolve behaviors based on empirical data, such as from sensor data or databases...
techniques to model a wide range of human activities. Mobile devices (e.g. smart phones) provide sufficient sensor data and calculation power to enable physical activity recognition to provide an estimation of the energy consumption during everyday life. Sensor-based activity recognition researchers believe that by empowering ubiquitous computers
Ubiquitous computing
Ubiquitous computing is a post-desktop model of human-computer interaction in which information processing has been thoroughly integrated into everyday objects and activities. In the course of ordinary activities, someone "using" ubiquitous computing engages many computational devices and systems...
and sensors to monitor the behavior of agents (under consent), these computers will be better suited to act on our behalf.
Levels of sensor-based activity recognition
Sensor-based activity recognition is a challenging task due to the inherent noisy nature of the input. Thus, statistical modeling has been the main thrust in this direction in layers, where the recognition at several intermediate levels is conducted and connected. At the lowest level where the sensor data are collected, statistical learning concerns how to find the detailed locations of agents from the received signal data. At an intermediate level, statistical inferenceStatistical inference
In statistics, statistical inference is the process of drawing conclusions from data that are subject to random variation, for example, observational errors or sampling variation...
may be concerned about how to recognize individuals' activities from the inferred location sequences and environmental conditions at the lower levels. Furthermore, at the highest level a major concern is to find out the overall goal or subgoals of an agent from the activity sequences through a mixture of logical and statistical reasoning. Scientific conferences where activity recognition work from wearable and environmental often appears are ISWC
International Symposium on Wearable Computers
The International Symposium on Wearable Computers or ISWC is one of the most prominent academic conferences on wearable computing and ubiquitous computing....
and UbiComp.
Sensor-based, multi-user activity recognition
Recognizing activities for multiple users using on-body sensors first appeared in the work by ORL using active badge systems in the early 90's. Other sensor technology such as acceleration sensors were used for identifying group activity patterns during office scenarios. Activities of Multiple Users in intelligent environments are addressed in Gu et al. In this work, they investigate the fundamental problem of recognizing activities for multiple users from sensor readings in a home environment, and propose a novel pattern mining approach to recognize both single-user and multi-user activities in a unified solution. Many interesting research topics can be spawn from this work.Vision-based activity recognition
It is a very important and challenging problem to track and understand the behavior of agents through videos taken by various cameras. The primary technique employed is computer vision. Vision-based activity recognition has found many applications such as human-computer interaction, user interface design, robot learning, and surveillance, among others.Scientific conferences where vision based activity recognition work often appears are ICCV and CVPR.
In vision-based activity recognition, a great deal of work has been done. Researchers have attempted a number of methods such as optical flow
Optical flow
Optical flow or optic flow is the pattern of apparent motion of objects, surfaces, and edges in a visual scene caused by the relative motion between an observer and the scene. The concept of optical flow was first studied in the 1940s and ultimately published by American psychologist James J....
, Kalman filtering, hidden Markov model
Markov model
In probability theory, a Markov model is a stochastic model that assumes the Markov property. Generally, this assumption enables reasoning and computation with the model that would otherwise be intractable.-Introduction:...
s, etc., under different modalities such as single camera, stereo, and infrared. In addition, researchers have considered multiple aspects on this topic, including single pedestrian tracking, group tracking, and detecting dropped objects.
Levels of vision-based activity recognition
In vision-based activity recognition, the computational process is often divided into four steps, namely human detection, human tracking, human activity recognition and then a high-level activity evaluation.Activity recognition through logic and reasoning
Logic-based approaches keep track of all logically consistent explanations of the observed actions. Thus, all possible and consistent plans or goals must be considered. Kautz provided a formal theory of plan recognition. He described plan recognition as a logical inference process of circumscription. All actions, plans are uniformly referred to as goals, and a recognizer's knowledge is represented by a set of first-order statements called event hierarchy encoded in first-order logic, which defines abstraction, decomposition and functional relationships between types of events.Kautz's general framework for plan recognition has an exponential time complexity in worst case, measured in the size of input hierarchy. Lesh and Etzioni went one step further and presented methods in scaling up goal recognition to scale up his work computationally. In contrast to Kautz's approach where the plan library is explicitly represented, Lesh and Etzioni's approach enables automatic plan-library construction from domain primitives. Furthermore, they introduced compact representations and efficient algorithms for goal recognition on large plan libraries.
Inconsistent plans and goals are repeatedly pruned when new actions arrive. Besides, they also presented methods for adapting a goal recognizer to handle individual idiosyncratic behavior given a sample of an individual's recent behavior. Pollack et al. described a direct argumentation model that can know about the relative strength of several kinds of arguments for belief and intention description.
A serious problem of logic-based approaches is their inability or inherent infeasibility to represent uncertainty. They offer no mechanism for preferring one consistent approach to another and incapable of deciding whether one particular plan is more likely than another, as long as both of them can be consistent enough to explain the actions observed. There is also a lack of learning ability associated with logic based methods.
Activity recognition through probabilistic reasoning
Probability theory and statistical learning models are more recently applied in activity recognition to reason about actions, plans and goals.Plan recognition can be done as a process of reasoning under uncertainty, which is convincingly argued by Charniak and Goldman. They argued that any model that does not incorporate some theory of uncertainty reasoning cannot be adequate. In the literature, there have been several approaches which explicitly represent uncertainty in reasoning about an agent's plans and goals.
Using sensor data as input, Hodges and Pollack designed machine learning-based systems for identifying individuals as they perform routine daily activities such as making coffee. Intel Research (Seattle) Lab and University of Washington at Seattle have done some important works on using sensors to detect human plans. Some of these works infer user transportation modes from readings of radio-frequency identifiers (RFID) and global positioning systems (GPS).
Wi-Fi-based activity recognition
When activity recognition is performed indoors and in cities using the widely available Wi-FiWi-Fi
Wi-Fi or Wifi, is a mechanism for wirelessly connecting electronic devices. A device enabled with Wi-Fi, such as a personal computer, video game console, smartphone, or digital audio player, can connect to the Internet via a wireless network access point. An access point has a range of about 20...
signals and 802.11 access points, there is much noise and uncertainty. These uncertainties are modeled using a dynamic Bayesian network
Bayesian network
A Bayesian network, Bayes network, belief network or directed acyclic graphical model is a probabilistic graphical model that represents a set of random variables and their conditional dependencies via a directed acyclic graph . For example, a Bayesian network could represent the probabilistic...
model by Yin et al. A multiple goal model that can reason about user's interleaving goals is presented by Chai and Yang, where a deterministic state transition model is applied. A better model that models the concurrent and interleaving activities in a probabilistic approach is proposed by Hu and Yang. A user action discovery model is presented by Yin et al., where the Wi-Fi signals are segmented to produce possible actions.
A fundamental problem in Wi-Fi-based activity recognition is to estimate the user locations. Two important issues are how to reduce the human labelling effort and how to cope with the changing signal profiles when the environment changes. Yin et al. dealt with the second issue by transferring the labelled knowledge between time periods. Chai and Yang proposed a hidden Markov model-based method to extend labelled knowledge by leveraging the unlabelled user traces. J. Pan et al. propose to perform location estimation through online co-localization, and S. Pan et al. proposed to apply multi-view learning for migrating the labelled data to a new time period.
Data mining based approach to activity recognition
Different from traditional machine learning approaches, an approach based on data mining has been recently proposed.In the work of Gu et al., the problem of activity recognition is formulated as a pattern-based classification problem. They proposed a data mining approach based on discriminative patterns which describe significant changes between any two activity classes of data to recognize sequential, interleaved and concurrent activities in a unified solution.
Gilbert et al use 2D corners in both space and time. These are grouped spatially and temporally using a hierarchical process, with an increasing search area. At each stage of the hierarchy, the most distinctive and descriptive features are learned efficiently through data mining (Apriori rule).
Labs in the world
- Martha Pollack's research group
- Prof Qiang Yang's research group
- RSE Lab @ University of Washington, leading by Dieter Fox
- Fraunhofer IGD Lab for Ambient Intelligence
- Tao Gu's Advanced Network System Lab at University of Southern Denmark
- Jeffrey Junfeng Pan's Sensor-based Localization and Tracking Project
- Ajou University CUSLAB Vision-based Activity Awareness
- Tanzeem Choudhury's People-Aware Computing (PAC) Group
- Computer Vision and Multimodal Computing Group at MPI INF
- Wearable Computing Lab at ETH Zurich
- The BehaviorScope Project at ENALAB - Yale
- The Embedded Sensing Systems group at TU DarmstadtDarmstadt University of TechnologyThe Technische Universität Darmstadt, abbreviated TU Darmstadt, is a university in the city of Darmstadt, Germany...
- WSU CASAS Smart Home Project
- DLR Institute for Communications and Navigation Activity Recognition Project
Related conferences
See also
- PlanningPlanningPlanning in organizations and public policy is both the organizational process of creating and maintaining a plan; and the psychological process of thinking about the activities required to create a desired goal on some scale. As such, it is a fundamental property of intelligent behavior...
- Naive Bayes classifierNaive Bayes classifierA naive Bayes classifier is a simple probabilistic classifier based on applying Bayes' theorem with strong independence assumptions...
- Support vector machines
- Hidden Markov modelHidden Markov modelA hidden Markov model is a statistical Markov model in which the system being modeled is assumed to be a Markov process with unobserved states. An HMM can be considered as the simplest dynamic Bayesian network. The mathematics behind the HMM was developed by L. E...
- Conditional random fieldConditional random fieldA conditional random field is a statistical modelling method often applied in pattern recognition.More specifically it is a type of discriminative undirected probabilistic graphical model. It is used to encode known relationships between observations and construct consistent interpretations...