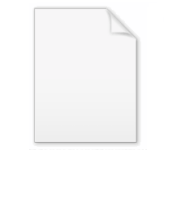
Neyer d-optimal test
Encyclopedia
The Neyer D-Optimal Test is one way of analyzing a sensitivity test of explosives as described by Barry T. Neyer in 1994. This method has replaced the earlier Bruceton analysis
or "Up and Down Test" that was devised by Dixon and Mood in 1948 to allow computation with pencil and paper. Samples are tested at various stimulus levels, and the results (response or no response) noted. The Neyer Test guides the experimenter to pick test levels that provide the maximum amount of information. Unlike previous methods that have been developed, this method requires the use of a computer program to calculate the test levels.
Although not directly related to the test method, the likelihood ratio analysis
method is often used to analyze the results of tests conducted with the Neyer D-Optimal test. The combined test and analysis methods are commonly known as the Neyer Test.
Sensitivity tests are not limited to testing explosives. Similar analysis is used to study the fracture toughness of materials (e.g. if a heavy bottle of liquid detergent will split open when it falls), drug efficacy (i.e. what dose of a drug is sufficient to cure the disease), avian toxicology (i.e. how much contaminated seed is sufficient to cause birds to die), and many other subjects.
Dror and Steinberg (2008) suggest another experimental design method which is more efficient then Neyer's, by enabling the usage of a D-optimal design criterion from the outset of the experiment. Furthermore, their method is extended to deal with situations which are not handled by previous algorithms, including extension from fully sequential designs (updating the plan after each observation) to group-sequential designs (any partition of the experiment to blocks of numerous observations), from a binary response ("success" or "failure") to any generalized linear model, and from the univariate case to the treatment of multiple predictors (such as designing an experiment to test a response in a medical treatment where the experimenters changes doses of two different drugs).
Bruceton analysis
A Bruceton Analysis is one way of analyzing a sensitivity test of explosives as described originally by Dixon and Mood in 1948. Also known as the "Up and Down Test," a Bruceton analysis relies upon two parameters: first stimulus and step size. A stimulus is provided to the sample, and the results...
or "Up and Down Test" that was devised by Dixon and Mood in 1948 to allow computation with pencil and paper. Samples are tested at various stimulus levels, and the results (response or no response) noted. The Neyer Test guides the experimenter to pick test levels that provide the maximum amount of information. Unlike previous methods that have been developed, this method requires the use of a computer program to calculate the test levels.
Although not directly related to the test method, the likelihood ratio analysis
Likelihood-ratio test
In statistics, a likelihood ratio test is a statistical test used to compare the fit of two models, one of which is a special case of the other . The test is based on the likelihood ratio, which expresses how many times more likely the data are under one model than the other...
method is often used to analyze the results of tests conducted with the Neyer D-Optimal test. The combined test and analysis methods are commonly known as the Neyer Test.
Sensitivity tests are not limited to testing explosives. Similar analysis is used to study the fracture toughness of materials (e.g. if a heavy bottle of liquid detergent will split open when it falls), drug efficacy (i.e. what dose of a drug is sufficient to cure the disease), avian toxicology (i.e. how much contaminated seed is sufficient to cause birds to die), and many other subjects.
Dror and Steinberg (2008) suggest another experimental design method which is more efficient then Neyer's, by enabling the usage of a D-optimal design criterion from the outset of the experiment. Furthermore, their method is extended to deal with situations which are not handled by previous algorithms, including extension from fully sequential designs (updating the plan after each observation) to group-sequential designs (any partition of the experiment to blocks of numerous observations), from a binary response ("success" or "failure") to any generalized linear model, and from the univariate case to the treatment of multiple predictors (such as designing an experiment to test a response in a medical treatment where the experimenters changes doses of two different drugs).