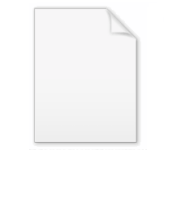
Lindley's paradox
Encyclopedia
Lindley's paradox is a counterintuitive situation in statistics
in which the Bayesian
and frequentist approaches to a hypothesis testing problem give opposite results for certain choices of the prior distribution. The problem of the disagreement between the two approaches was discussed in Harold Jeffreys
' textbook; it became known as Lindley's paradox after Dennis Lindley
called the disagreement a paradox
in a 1957 paper.
H0, the result of an experiment x, and a prior distribution that favors H0 weakly. Lindley's paradox occurs when
These results can happen at the same time when the prior distribution is the sum of a sharp peak at H0 with probability p and a broad distribution with the rest of the probability 1 − p. It is a result of the prior having a sharp feature at H0 and no sharp features anywhere else.
) of male births is thus 49,581/98,451 ≈ 0.5036. We are interested in testing whether the true proportion (
) is 0.5. That is, our null hypothesis is
and the alternative is
.
and
, the latter uniformly distributed between 0 and 1. The prior distribution is thus a mixture of point mass 0.5 and a uniform distribution
. The number of male births is a binomial variable with mean
and variance
, where
is the total number of births (98,451 in this case). Because the sample size is very large, and the observed proportion is far from 0 and 1, we can use a normal approximation for the distribution of
. Because of the large sample, we can approximate the variance as
. The posterior probability is
.
So we find that there is not enough evidence to reject
.
.
Because we are performing a two-sided test (we would have been equally surprised if we had seen 48,870 boy births, i.e.
), the p-value is
, which is lower than the significance level of 5%. Therefore, we reject
.
The two approaches—the Bayesian and the frequentist—are in conflict, and this is the paradox.
Statistics
Statistics is the study of the collection, organization, analysis, and interpretation of data. It deals with all aspects of this, including the planning of data collection in terms of the design of surveys and experiments....
in which the Bayesian
Bayesian inference
In statistics, Bayesian inference is a method of statistical inference. It is often used in science and engineering to determine model parameters, make predictions about unknown variables, and to perform model selection...
and frequentist approaches to a hypothesis testing problem give opposite results for certain choices of the prior distribution. The problem of the disagreement between the two approaches was discussed in Harold Jeffreys
Harold Jeffreys
Sir Harold Jeffreys, FRS was a mathematician, statistician, geophysicist, and astronomer. His seminal book Theory of Probability, which first appeared in 1939, played an important role in the revival of the Bayesian view of probability.-Biography:Jeffreys was born in Fatfield, Washington, County...
' textbook; it became known as Lindley's paradox after Dennis Lindley
Dennis Lindley
Dennis Victor Lindley is a British statistician, decision theorist and leading advocate of Bayesian statistics.Dennis Lindley grew up in the south-west London suburb of Surbiton. He was an only child and his father was a local building contractor...
called the disagreement a paradox
Paradox
Similar to Circular reasoning, A paradox is a seemingly true statement or group of statements that lead to a contradiction or a situation which seems to defy logic or intuition...
in a 1957 paper.
Description of the paradox
Consider a null hypothesisNull hypothesis
The practice of science involves formulating and testing hypotheses, assertions that are capable of being proven false using a test of observed data. The null hypothesis typically corresponds to a general or default position...
H0, the result of an experiment x, and a prior distribution that favors H0 weakly. Lindley's paradox occurs when
- The result x is significant by a frequentist test, indicating sufficient evidence to reject H0, say, at the 5% level, and
- The posterior probabilityPosterior probabilityIn Bayesian statistics, the posterior probability of a random event or an uncertain proposition is the conditional probability that is assigned after the relevant evidence is taken into account...
of H0 given x is high, say, 95%, indicating strong evidence that H0 is in fact true.
These results can happen at the same time when the prior distribution is the sum of a sharp peak at H0 with probability p and a broad distribution with the rest of the probability 1 − p. It is a result of the prior having a sharp feature at H0 and no sharp features anywhere else.
Numerical example
We can illustrate Lindley's paradox with a numerical example. Let's imagine a certain city where 49,581 boys and 48,870 girls have been born over a certain time period. The observed proportion (



Bayesian approach
We have no reason to believe that the proportion of male births should be different from 0.5, so we assign prior probabilities








So we find that there is not enough evidence to reject

Frequentist approach
Using the normal approximation above, the upper tail probability is
Because we are performing a two-sided test (we would have been equally surprised if we had seen 48,870 boy births, i.e.



The two approaches—the Bayesian and the frequentist—are in conflict, and this is the paradox.